AI Governance Framework & Best Practices
Regulations are necessary, especially when AI-driven decisions impact our daily lives, ensuring citizens’ well-being while fostering professional innovation.
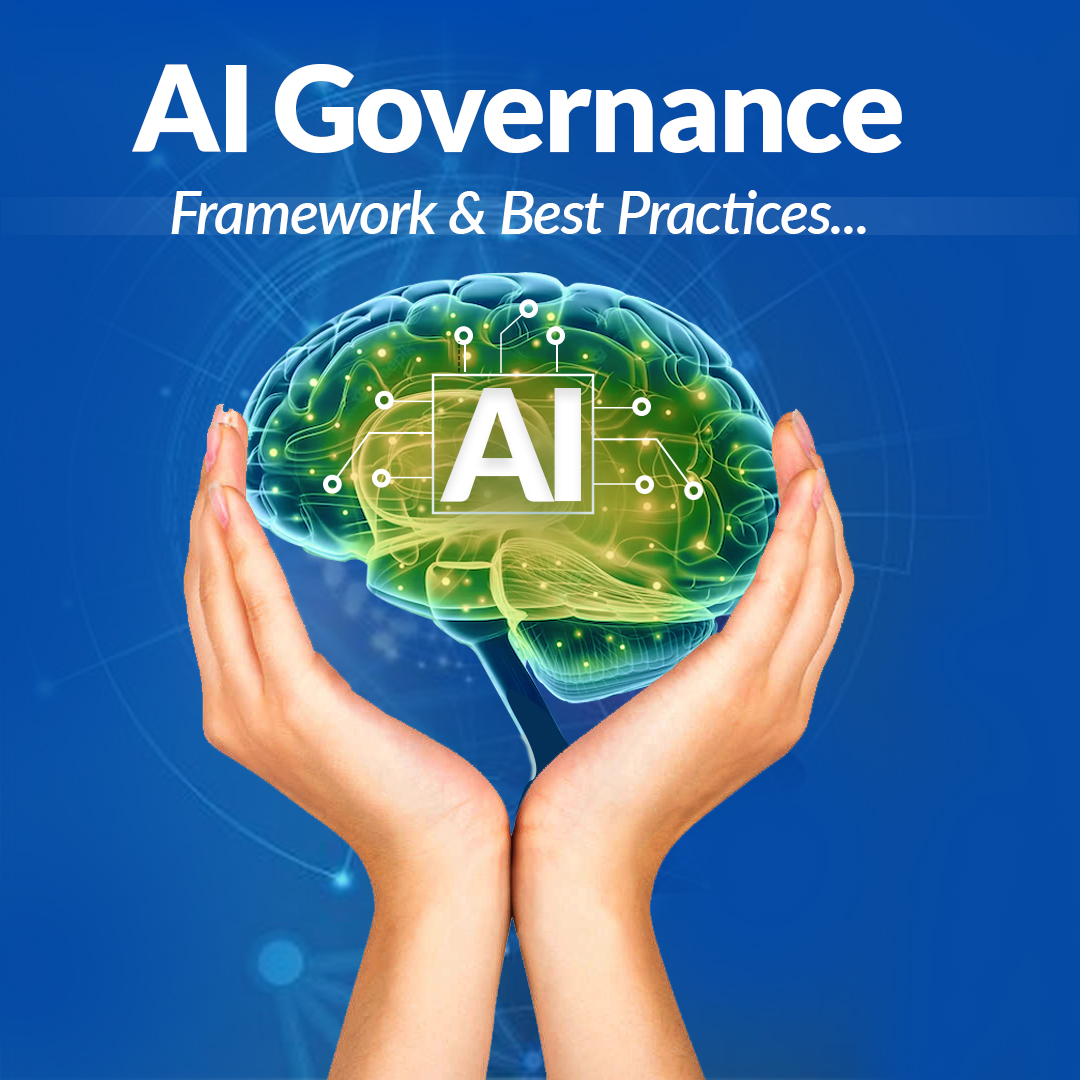
“Effective AI governance methods save millions of dollars by ensuring data security and quality. Poor data quality causes an annual loss of $15 million, while the average cost of a data breach is $3.92 million. AI governance practices help organizations mitigate risks, prevent breaches, and generate significant financial savings.”- Gartner
What Exactly is AI Governance?
AI governance refers to the frameworks, regulations, and guidelines that govern the development, adoption, and application of AI technology. It has gained significant attention as governments and organizations recognize the importance of responsible AI practices that uphold moral values, transparency, and societal benefits. Effective AI governance addresses critical issues like privacy, bias, justice, accountability, and safety. Businesses must stay informed about emerging laws and policies to ensure compliance in their AI systems’ creation and deployment. This involves integrating ethical considerations into AI development processes, conducting regular audits and risk assessments, and fostering transparency by providing justifications for AI-driven decisions.
Furthermore, businesses should consider the potential impact of AI on employees and customers, implementing policies to mitigate adverse effects and minimize risks. By adhering to robust AI governance best practices, organizations can navigate the ethical landscape and promote the responsible use of AI for the betterment of society.
AI Governance Strategy
Strong executive support is crucial for any AI Governance strategy, ensuring data quality, security, and administration. Each team should take ownership of the data, models, and tasks they manage, fostering a culture of continuous integration and data ownership. Effective top-down communication and recognition are necessary for the bottom-up strategy’s implementation. In many organizations, data scientists and business intelligence teams have historically prioritized speed over quality, unlike traditional software development practices. However, the landscape has evolved, and now there is a growing emphasis on quality in data products. Rather than stifling innovation, governance should foster it, creating an environment that encourages both innovation and responsible practices.
AI Governance Framework
AI Ethics & Humanity: AI Ethics ensures AI systems prioritize human well-being, privacy, and consent. Recommendation engines must avoid bias and harmful content, while human oversight is essential in sensitive domains. Fairness, privacy, and ethical guidelines should be central to AI Governance in categorization and generative engines, promoting inclusivity and diverse perspectives. Respecting human dignity and upholding ethical standards in content generation fosters trust and a positive societal impact. Striking this balance between AI capabilities and human values is crucial for AI’s responsible implementation.
AI Responsibility: AI Responsibility demands clear accountability for AI systems’ development and deployment. Transparent documentation, bias detection, and fairness mechanisms are crucial. Users must control content filtering, and human oversight ensures accountability. Categorization counters stereotypes with bias detection and human monitoring. Generative engines generate controlled outputs with user feedback. This fosters transparency, trust, and positive societal impact, emphasizing responsible AI practices in the AI Governance paradigm.
AI Risk: The AI Governance framework takes AI Risk seriously. The recommendation engine’s potential risks include filter bubbles, biased reinforcement, and susceptibility to manipulation and exploitation. To address these challenges, the framework employs advanced bias detection and mitigation techniques. In categorization, the risks of mislabeling and misinterpretation are managed through continuous review and improvement processes. Additionally, the Generative Engine’s risks, like offensive content generation and identity-related threats, are countered with strict oversight, content filtering, and robust measures against impersonation and identity theft. Proactive risk management ensures responsible AI integration, fostering positive societal outcomes.
AI Trust: Trust in AI systems is essential for widespread adoption. Transparency, explainability, and accountability foster trust. Users should understand how AI operates, aided by explainable AI techniques. Data privacy and protection are crucial for user trust. AI Governance ensures transparency and accountability in Recommendation, Categorization, and Generative Engines. Trust is built through reproducible outcomes, unbiased categorization, and safeguarding against manipulation. Users rely on AI’s reliability and dependability, cultivating a sense of trustworthiness in AI applications.
AI Scale & AI Ops: AI Scale refers to handling large data, users, and interactions effectively. Recommendation, categorization, and generative engines require robust infrastructure, optimized algorithms, and hardware for smooth operations. Ensuring fair competition and preventing AI power concentration is crucial. AI Ops involves managing AI systems throughout their lifecycle, including model training, testing, deployment, and maintenance. Regular updates and user feedback are essential for accuracy and reliability. AI Scale & AI Ops are vital for AI Governance, ensuring efficient and effective operations. Scalable model engines, real-time data collection, and fine-tuning parameters enhance performance and adaptability. This comprehensive approach fosters agility, responsiveness, and reliability in AI systems.
Further Read: Why do Businesses Need an AI Strategy to Succeed
AI Governance Best Practices
Organizations should consider the following best practices to establish a robust AI governance framework:
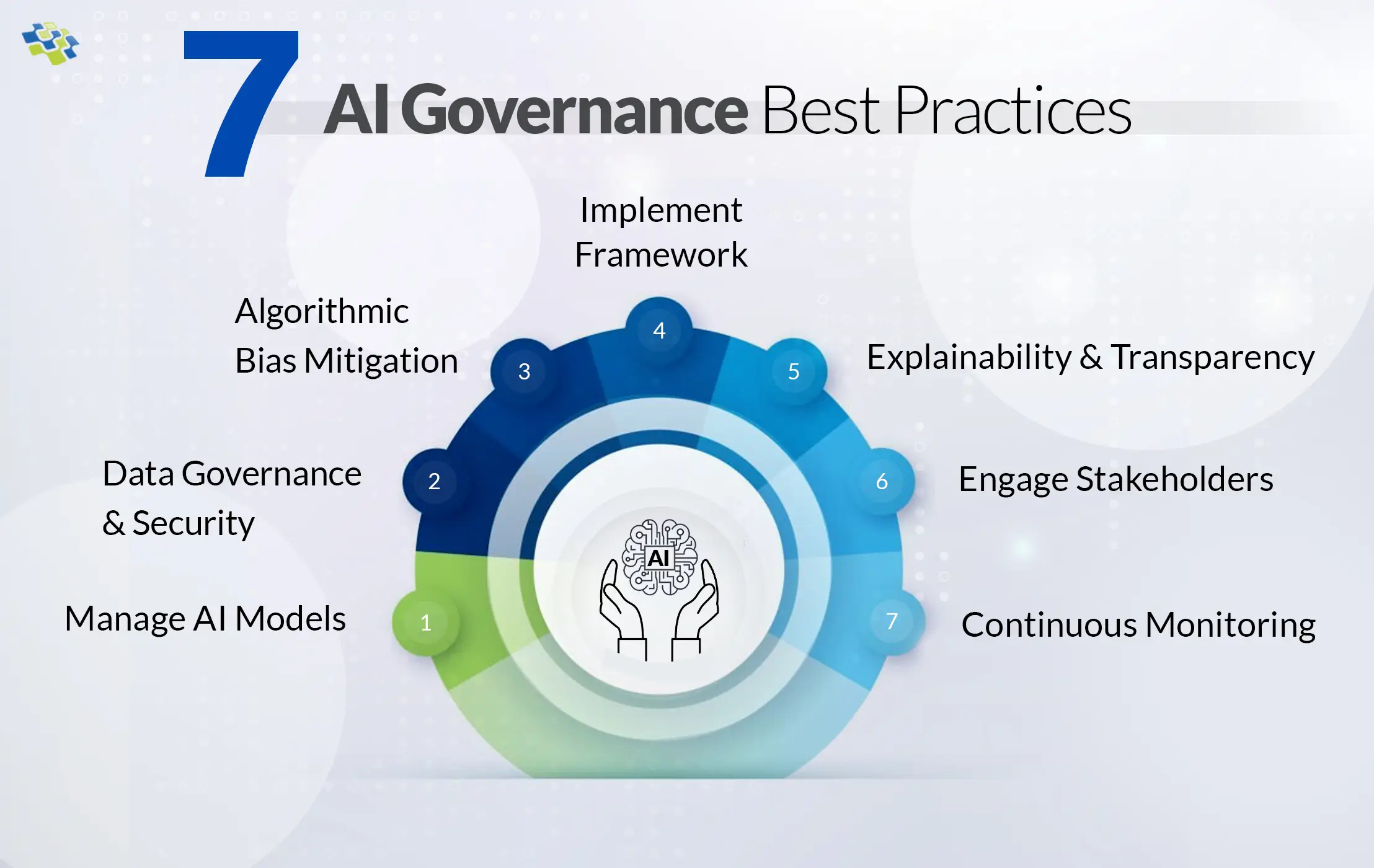
1. Manage AI Models
Continuous monitoring, model updates, and ongoing testing are important measures organizations can take to ensure the performance of AI models. Over time, AI models can experience deterioration, leading to undesirable outcomes. By conducting regular testing, organizations can detect and address issues like model drift, ensuring the system remains reliable. Additionally, periodic model refreshes help incorporate new data and insights, enhancing accuracy and relevance. Continuous monitoring allows for real-time assessment, enabling timely interventions and maintaining the system’s intended functionality.
2. Data Governance & Security
In the context of artificial intelligence, enterprises often gather and utilize sensitive consumer data, ranging from demographics and social media activity to geographical information and online shopping patterns. To uphold the integrity of AI system outcomes and adhere to data security and privacy laws, it is imperative to establish robust data security and governance standards. By developing AI-specific data governance and security rules, organizations can effectively mitigate the risks associated with data theft or exploitation. This proactive approach not only safeguards sensitive consumer information but also fosters trust, ensuring responsible AI governance while leveraging the valuable insights derived from consumer data.
3. Algorithmic Bias Mitigation
Unintentional cognitive biases and negative associations inherent in humans often permeate into AI systems, leading to unfair biases that can impact hiring practices or customer service standards based on demographics such as gender or race. To address this challenge, appropriate pre- and post-processing techniques, such as option-based categorization, can be employed to identify and rectify biases by assigning corrective weights. Adversarial debiasing, an in-processing method, involves developing a secondary model to predict the sensitive features causing bias in the initial model. Additionally, tools like what-if analysis enable interactive visual examination, stress testing, and identification of limitations and blind spots, aiding in minimizing biases. These measures promote fairness and equity in AI systems, ensuring they operate without unjust discrimination.
4. Implement Frameworks
AI governance frameworks must be implemented with robust safeguards to ensure compliance. This includes establishing a reporting structure that extends to senior leadership, fostering an organizational culture that prioritizes AI ethics, effectively communicating findings and insights, conducting regular audits, and clearly defining roles and responsibilities for managing AI systems. By creating a reporting mechanism that reaches senior leadership, organizations can ensure accountability and prompt action. Educating staff members on AI ethics promotes awareness and responsible practices. Routine audits help identify potential issues and ensure compliance, while clear role definitions streamline decision-making and oversight processes. These measures collectively strengthen the implementation of AI governance frameworks and promote responsible AI practices throughout the organization.
5. Explainability & Transparency
Developers frequently overlook the crucial component of improving model transparency in their persistent pursuit of accuracy. Initially, artificial intelligence (AI) systems were treated as impenetrable “black boxes,” with minimal effort to reveal their inner workings beyond input and output. However, the rise of accountability concerns in automated decision-making has led to regulatory measures like the General Data Protection Regulation (GDPR), which grants the right to an explanation. Resolving conflicts arising from AI-powered solutions requires mediators to grasp the underlying causes and assign responsibility appropriately. Several methods can bolster explainability in AI systems. Proxy modeling, such as using decision trees, aids in understanding complex models, while the “interpretability by design” approach emphasizes constructing the network from smaller, more intelligible components. By prioritizing model transparency alongside accuracy, organizations can foster responsible and trustworthy AI systems.
6. Engage Stakeholders
Creating a comprehensive and inclusive AI governance framework requires the participation of management, personnel, customers, partners, information security experts, and regulators. By involving multiple perspectives, organizations can create a more robust framework that addresses a wide range of issues. This collaborative approach ensures that the AI governance framework considers various viewpoints, incorporates different expertise, and incorporates the needs and concerns of all stakeholders involved. Engaging stakeholders throughout the design and implementation process promotes transparency, accountability, and a shared understanding of the ethical and practical considerations surrounding AI systems.
7. Continuous Monitoring
Maintaining ethical standards requires establishing procedures for continuous monitoring and auditing of AI systems. This entails conducting regular evaluations of data sources, assessing model behavior, and monitoring performance metrics. Ongoing monitoring allows organizations to detect and address any potential issues, such as biases, data drift, or system degradation. Regular audits provide opportunities to verify compliance with relevant regulations, identify areas for improvement, and ensure that the AI systems continue to operate as intended. By incorporating these monitoring and auditing practices, organizations can maintain the integrity, fairness, and effectiveness of their AI systems over time.
Further Read: Improving ML Model Performance: 5 Key Steps You Should Follow
AI Governance Challenges
- Regulatory Variations: Adhering to diverse regulatory frameworks across different countries poses a challenge in maintaining consistent AI governance standards. Organizations must navigate the complexities of complying with various requirements, privacy regulations, and data protection laws.
- Third-Party Management: Managing third-party technologies introduces complexities in ensuring compliance, transparency, and accountability. Organizations need a clear and well-defined approach to assess and monitor the governance practices of external vendors or partners whose AI systems are integrated into their operations.
- Customization & Discretion: Determining what aspects of AI governance should be standardized and mandatory versus discretionary and tailored to specific use cases can be challenging. Striking the right balance between uniformity and flexibility requires careful consideration and a deep understanding of the specific industry, context, and regional variations.
- Training & Awareness: Establishing a comprehensive training and awareness program across the three Lines of Defence (LoDs) is crucial for effective AI governance. This includes educating employees at all levels about ethical considerations, bias mitigation, data privacy, and the responsible use of AI technologies to ensure alignment with organizational values and regulatory requirements.
Conclusion
A robust AI governance framework is of utmost importance. It involves establishing ethical guidelines and ensuring transparent and accountable AI use. Building trust and promoting public awareness requires open discussion of AI’s limitations and potential threats. Businesses are aware of AI’s transformative potential for staying competitive, despite constant concerns about its influence on the job market. Striking a balance between embracing AI as a valuable tool and upholding the importance of human judgment is critical. Adopting AI responsibly requires building a secure ecosystem based on guiding principles. We can realize AI’s enormous potential while defending user interests and advancing societal well-being by proactively addressing its governance.
As we navigate the AI-powered future, a strong focus on responsible governance is key to shaping a prosperous and inclusive digital age. Join us at NextGen Invent, where we develop AI-enabled solutions for the modern world, working together to define a winning AI strategy.
Contact us today to harness the potential of AI responsibly, driving your business competitiveness while safeguarding user interests and societal well-being, and embark on your AI journey with trust and integrity.
Stay In the Know
Get Latest updates and industry insights every month.