Artificial-intelligence, Digital-transformation
Decision Making Framework for AI based Product businesses
NextGen Invent Corporation has built a decision-making framework for entrepreneurs and business innovators. To address the following questions that arise when you have a business idea using AI and workflow enhancement.
- I have an AI business idea, but what’s next?
- What will increase the adoption of my AI solutions?
- How can I make sure that my data science team is going to build an accurate model?
- What are the success factors and forces that go into making my AI model effective?
- What are the risks associated with my AI product/service adoption and sales cycle?
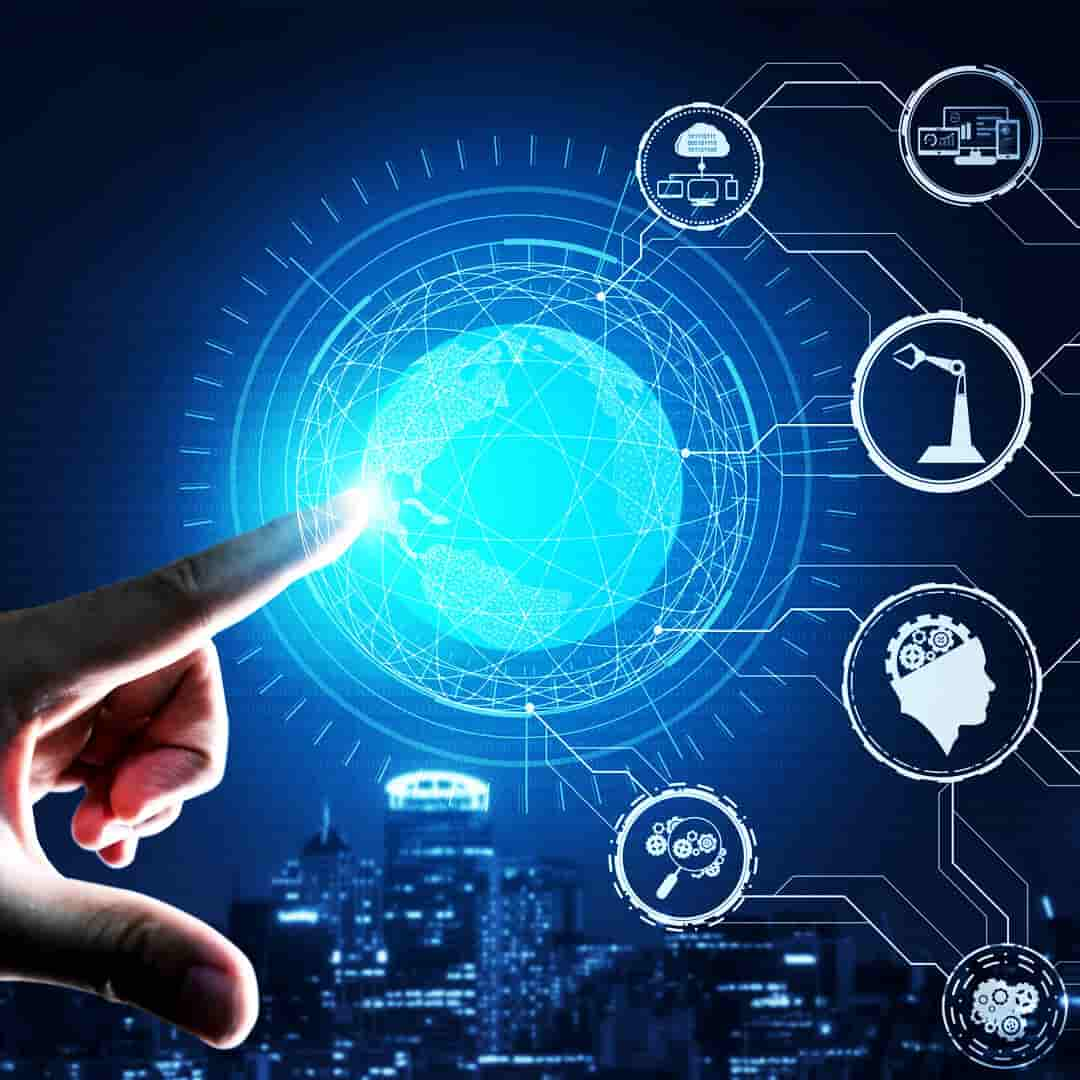
An agile business framework is key to avoiding expensive mistakes and ensuring a positive business outcome. Whether the idea is to start a new AI-based product/service business or to transform an existing product, a business framework plays a crucial role.
Most entrepreneurs would agree that they had a million-dollar AI-based product idea but were unable to bring it to reality because they were unaware of the direction they were supposed to take.
Therefore, having a solid business framework to guide decision-making processes is an important tool.
NextGen Invent’s decision-making framework is based on the experience gathered from over three hundred AI model developments and from interviews with 50+ executives and 20+ successful entrepreneurs. The proposed business framework highlights five key principles that play a vital role in determining the course of your AI business:
An entrepreneur should consider these five key principles to be successful in defining an AI/ML/Deep Learning model for bringing innovative products and services to the market. Let’s understand each key principle in detail.
Be the Model User
- If you are an entrepreneur with a goal to launch an AI model-based product or service, you should imagine yourself to be the model user. Define your expectations from the model. This will help you determine the input format, the manner in which it is processed.
- The output is given to your users.
- After receiving the input, you must understand, as a model, the success rate of the output provided. In other words, ask yourself how knowledgeable you should be as a model used to provide the input to utilize model output.
- You should make sure that your model output is relevant to users’ needs based on vast data knowledge.
- If you are an entrepreneur and not a data scientist then this step brings you closer to understanding your AI product and services at a deeper level.
- This step will help you in asking the right questions as well.
Entry barrier
- In Michael Porter’s five forces, the most critical force for the success of a product or service is Entry Barrier. In most cases, the entry barrier for an AI model comes from its input data.
- Most companies are moving towards gathering Real World Data, gaining exclusive data rights, and improving data quality.
- 360-degree data approach along with strong data quality standards are crucial ingredients in making a unique model.
- value proposition and an entry barrier to your business.
- Once your model has an entry barrier and value to the customer, it is important yet difficult to maintain that position.
- Continuous learning, additional data to increase model intelligence, and expanding strategic partnerships/distribution channels may help you in staying ahead of curve.
Ethics
- “Ethics is knowing the difference between what is right and what is wrong”.
- Unfortunately, there will always be a gray area as law and social awareness will change over time.
- As an entrepreneur, the boundaries you set and the decisions you make in regard to ethics.
- Having an AI model with high standards for data privacy will tend to provide sustainable products and services.
Model Risk
Like all other businesses, AI-based products and services have their own set of unique risks. We can categorize these risks as follows:
1. Trust
An AI model is seen as a black box, i.e. input is provided to receive an output, but is it very hard to gain insight into what happened inside the box. Even though a model is using several black-box components one should spend extensive time in quality test data and scenarios. Trust in a model normally comes from well-documented benefits achieved from the AI model in real scenarios.
2. Future Redundancy
Due to ever-changing and fast-paced technology, the model that is considered worthy today might end up being redundant in the future. Therefore, plan to periodically audit and analyze your model’s performance in different scenarios.
3. Data Compliance
In most cases, the data used for your AI model has to follow a set of rules and regulations like HIPAA, GDPR, etc. As these laws are changing, a model that is compliant today may not be in the future.
Staying ahead of the curve and using technologies such as federated learning are commonly implemented approaches in these cases.
Model Adoption
- This is where an entrepreneur or other business executives spend most of their time.
- Executives should keep working on how to increase model value and make it easy to use.
- The adoption of AI is one of the hardest dimensions in any organization.
- It must adopt at all levels of the organization for it to be successful.
- Entrepreneurs and executives should be aware of the above business framework and related key principles.
- We would love to know how this framework has helped your business.
- To get more information or discuss your AI initiative, please click here.
Stay In the Know
Get Latest updates and industry insights every month.