Adoption of Generative AI in Healthcare & Life Sciences
To have a deep discussion on this, the NextGen Invent team evaluated existing and upcoming solutions, analyzing various use cases across healthcare and life sciences. Our podcast, featuring esteemed company founder Deepak Mittal and AI expert Partha Anbil, shed light on the mysteries surrounding Generative AI in healthcare and life sciences.
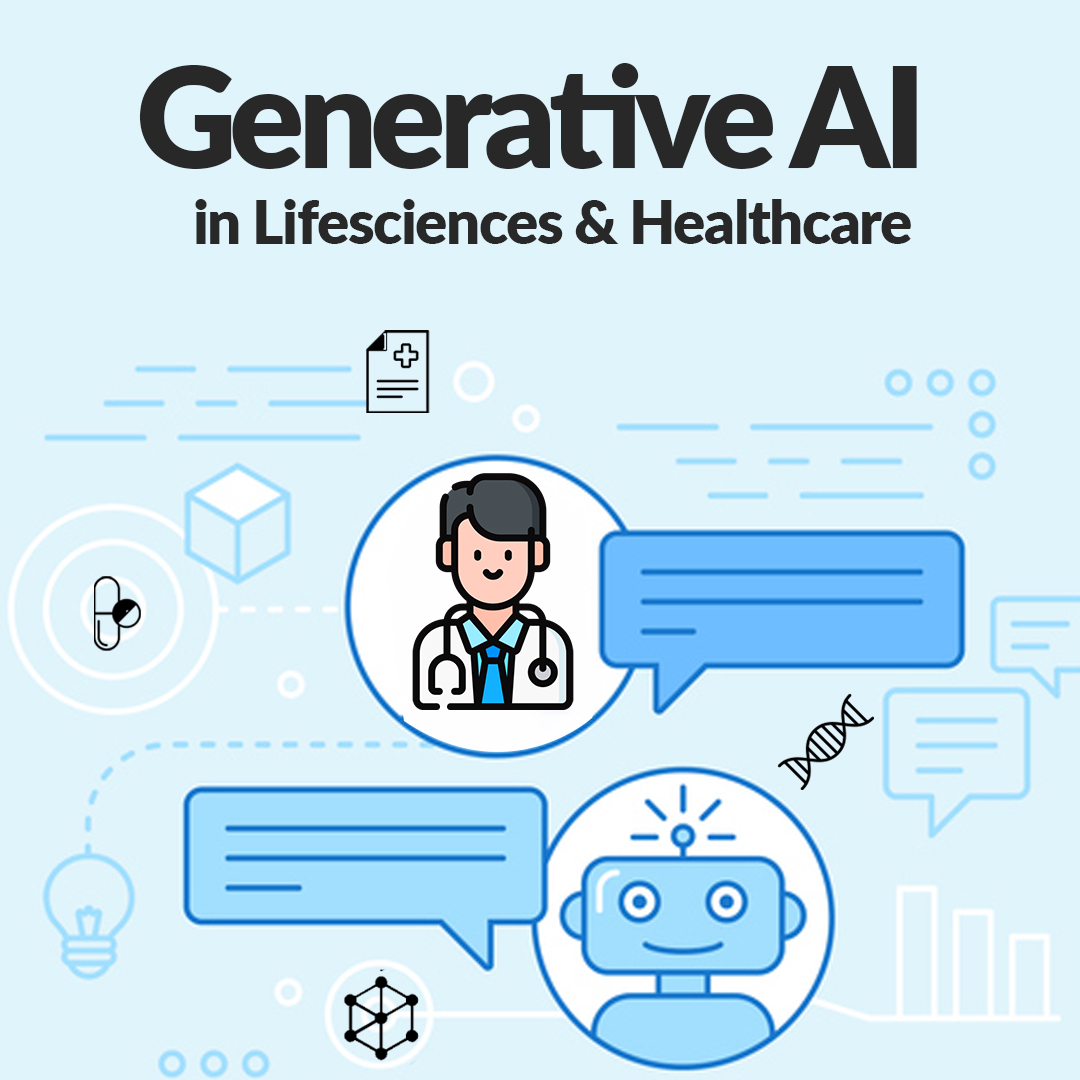
“The global market for Generative AI in the life sciences is projected to surge from USD 155.7 million in 2022 to USD 947 million by 2032, with a remarkable CAGR of 20.35% during the period from 2023 to 2032. This substantial growth reflects the increasing adoption and transformative potential of Generative AI in the industry.”- Market Research
Generative AI Use Cases in Healthcare & Life Sciences
In healthcare and life sciences, Generative AI employs deep learning neural networks inspired by the human brain’s structure. These networks consist of interconnected nodes arranged in layers, processing input data to generate content. The process begins by feeding the network with data such as medical images, patient records, or genetic sequences. Each node in the network processes the input data and passes it to the next layer, gradually refining the information. The final output is generated by the last layer, which could be diverse content like medical images, drug compounds, or even research papers. This technology’s flexibility enables it to create various formats, benefiting the industry in multiple ways.
Generative AI holds vast potential in healthcare and life sciences, facilitating medical image generation, personalized treatment plans, drug discovery, and even generating synthetic patient data for research purposes. By leveraging large-scale medical data analysis, this technology contributes to enhanced diagnostics, treatment accessibility, and fostering innovation for improved patient care and outcomes.
Generative AI plays a crucial role in healthcare and life sciences by fostering research and development, enhancing processes, and stimulating innovative ideas, contributing to transformative advancements and breakthroughs across industries.
1. Drug Discovery
Generative AI has emerged as a powerful tool to accelerate drug discovery, offering a transformative approach compared to the traditional lengthy and costly methods. By leveraging vast datasets of chemical structures and properties, Generative AI algorithms can rapidly produce novel drug compounds with potential therapeutic value. This expedites the identification of promising candidates for further evaluation in the laboratory. The process of drug discovery, which involves finding viable medications, can be significantly hastened as Generative AI sifts through a vast collection of substances to highlight prospective compounds. Through deep learning techniques, Generative AI efficiently creates virtual compounds, streamlining the pilot stages of drug research and enabling researchers to focus their efforts on the most promising candidates. This groundbreaking synergy between technology and pharmaceutical science holds the potential to revolutionize the development of new medications, benefiting patients and society.
2. Medical Chatbots
Generative AI has opened new possibilities in healthcare, enabling the development of medical chatbots that deliver personalized and accurate information to patients. One notable example is Babylon Health, which has successfully implemented a chatbot capable of gathering patient symptoms and leveraging Generative AI to offer tailored medical recommendations. This innovation has shown promising results, as studies indicate that 34% of patients prefer chatbot consultations for non-urgent medical issues. Moreover, according to Frost & Sullivan, AI can improve patient outcomes by 30% to 40% while reducing treatment costs by 50%. By harnessing the power of Generative AI, medical chatbots can efficiently analyse vast medical databases, quickly assimilate patient information, and offer individualized guidance to users, thereby enhancing accessibility and patient experience. The potential impact of this technology on healthcare is substantial, with estimates suggesting that AI-powered chatbots could save the healthcare industry $3.6 billion annually by 2023.
3. Personalized Treatment Plans
Generative AI has emerged as a groundbreaking approach in healthcare, enabling the development of individualized treatment plans by leveraging extensive patient data. A remarkable example of this is seen in Mayo Clinic’s research, where they successfully devised a deep learning algorithm that predicts post-surgery complications with impressive accuracy. This algorithm draws insights from vast volumes of patient data, allowing it to produce personalized treatment recommendations based on the specific risk factors identified for everyone. By providing tailored treatment plans, Generative AI not only enhances patient outcomes but also contributes to significant cost savings. Research estimates indicate that individualized treatment plans could lead to a 25% reduction in treatment costs. This exemplifies the immense potential of Generative AI in revolutionizing patient care and advancing the effectiveness of medical interventions.
4. Synthetic Gene Design
Generative AI has unlocked exciting possibilities in synthetic biology, particularly in the design of synthetic gene sequences. By harnessing the power of AI algorithms, researchers can efficiently create novel gene sequences for diverse applications, including the development of innovative biosynthetic pathways and the optimization of gene expression for biomanufacturing processes. One striking example comes from a study where Generative AI was employed to design synthetic gene circuits for microbial engineering. By the end of 2035, it is predicted that the global market for synthetic biology would have generated USD 288 billion in sales, expanding at a CAGR of 29.50%. By rapidly and accurately generating synthetic gene sequences, Generative AI is accelerating advancements in biotechnology, paving the way for new bio-based products, sustainable solutions, and groundbreaking breakthroughs in the fields of medicine, agriculture, and industrial biomanufacturing.
5. Telemedicine & Remote Patient Monitoring
Virtual assistants powered by Generative AI have revolutionized patient care by offering comprehensive support in health management. These advanced AI systems can efficiently handle tasks such as organizing health information, scheduling appointments, and facilitating seamless access to medical care. Moreover, virtual assistants extend their impact beyond administrative duties by remotely monitoring patients using data from wearables, sensors, and other monitoring devices. This real-time analysis equips medical professionals with immediate insights into a patient’s health status, enabling timely interventions and personalized care plans. The significance of this technology is evident in its potential to enhance patient outcomes and reduce healthcare costs. According to one study, RPM for individuals with heart failure saved healthcare expenses by $10,000 per patient-year.
6. Single-cell RNA Sequencing
Gene expression profiles can be examined at the individual cell level with single-cell RNA sequencing (scRNA-seq). However, the raw data obtained from scRNA-seq experiments often contain noise and undesired artifacts that can hinder accurate analysis and interpretation. Data denoising for scRNA-seq is a critical process aimed at removing these confounding factors, ensuring the generation of reliable and high-quality gene expression profiles for each cell. The impact of denoising scRNA-seq data is profound, as it directly influences the precise identification of cell types, differentiation trajectories, and other biologically significant insights. In this context, the application of generative models has shown great promise. By utilizing generative models, such as autoencoders or variational autoencoders, scRNA-seq data can be denoised effectively, leading to improved downstream analysis. The enhanced accuracy in cell-type identification and gene expression measurements facilitates a deeper understanding of cellular heterogeneity and complex biological processes, bringing us closer to unlocking crucial discoveries in various fields, including developmental biology, cancer research, and regenerative medicine.
7. Data Augmentation for Model Training
Generative models play a pivotal role in advancing the performance of AI models across critical tasks, such as medical imaging interpretation and precise diagnosis. By leveraging generative models, synthetic data can be produced to complement existing datasets, leading to significant improvements in model accuracy and robustness. Studies have demonstrated the efficacy of this approach, where the integration of synthetic data with real-world data has shown up to a 20% enhancement in the performance of medical image analysis algorithms. Also, according to Gartner, by 2024, 60% of data in AI and analytics projects will be synthetically generated. Furthermore, in drug discovery, generative models enable the creation of diverse virtual compounds, expanding the pool of potential drug candidates and accelerating the search for new medications. The use of synthetic data is particularly valuable in scenarios, where obtaining large, annotated datasets is challenging due to privacy concerns or the rarity of specific medical conditions. By harnessing the power of generative models to produce synthetic data, AI models can achieve greater generalization and proficiency, fostering breakthroughs in healthcare, research, and pharmaceutical advancements.
Further Read: An Executive’s Guide to Generative AI Revolution
Generative AI Challenges in in Healthcare & Life Sciences
Generative AI holds tremendous promise for life sciences and healthcare, but several challenges must be addressed before the technology can be fully realized:
1. Need for Large Datasets
Generative AI heavily relies on large datasets for effective learning, but challenges arise when such datasets are scarce or non-existent, as seen in some healthcare settings. The limited data availability in these domains can hinder the usefulness of Generative AI models for medical research, diagnostics, and treatment. To address this issue, exploring alternative approaches, such as data augmentation, transfer learning, and synthetic data generation, becomes imperative. Leveraging statistical techniques and small-scale datasets may help mitigate the data scarcity problem and promote the development of valuable Generative AI applications in healthcare.
2. Interpretability
The interpretability of Generative AI poses a significant challenge, particularly in healthcare, where understanding how the algorithm arrives at specific conclusions is crucial for trust and acceptance. The lack of transparency can hinder adoption and hinder the integration of AI-generated suggestions into clinical decision-making processes. Hence, developing explainable AI techniques and fostering transparency becomes paramount to gaining the trust and confidence of healthcare professionals in leveraging Generative AI in medical settings.
3. Model Validation & Interpretation
Generative AI models, such as consumer-grade LLMs like ChatGPT, can exhibit inaccuracies and unreliability due to their opaque and complex nature. In medical and clinical contexts, these models may “hallucinate” responses, presenting them with unwarranted certainty, an unacceptable characteristic. Furthermore, unless specifically designed for it, these AI models often lack coherent justifications or supporting data for their decisions or outputs. As a result, the potential risks and uncertainties associated with using such models in critical healthcare scenarios underscore the need for robustness, explainability, and caution in their deployment.
4. Data Quality
Generative AI and LLMs heavily rely on vast amounts of high-quality data for effective training and model improvement. In the life sciences, data is often plagued by inconsistencies, noise, incompleteness, and heterogeneity, presenting significant challenges. Moreover, life science data may be confidential or proprietary, limiting its accessibility. To harness the full potential of LLMs in the life science domain, businesses must prioritize ensuring access to diverse and reliable data sources. Addressing data quality issues and establishing appropriate data-sharing frameworks are crucial steps toward leveraging LLMs’ capabilities for advancements in life science research and applications.
How Can NextGen Invent Help?
As health organizations expand their applications, they can establish a solid foundation for adopting and implementing Generative AI technologies. Our developers employ a meticulous approach to comprehend your business objectives and create captivating; user-friendly Generative AI solutions tailored to your target audience’s needs.
- Define Generative AI Strategy: Our team provides expert guidance in formulating a customized Generative AI strategy for the healthcare and life sciences sectors. By closely aligning AI goals with the specific requirements and challenges of these industries, we ensure that the implemented solutions effectively contribute to improved healthcare outcomes and scientific advancements.
- Data Management: We excel in managing and optimizing data for Generative AI applications. Our meticulous approach ensures data security, adherence to industry standards, and effective data handling procedures. We enable strong and trustworthy AI-driven insights for improvements in healthcare and the life sciences by upholding the integrity and confidentiality of data.
- Develop Models: Our experienced team specializes in creating and refining cutting-edge generative AI models that are specifically suited to specific research and healthcare concerns. We provide healthcare professionals and researchers with AI solutions that provide insightful data and help to accelerate medical advancements by utilizing cutting-edge methodologies and domain expertise.
- Train Models: With the use of top-notch datasets, we use strict model training methodologies. This strategy improves the precision and efficacy of our Generative AI models, enabling them to produce insightful data with great accuracy for applications in the healthcare and life sciences.
- Continuous Improvement & Automation: Our developers place a high priority on automation and ongoing refinement to continuously improve our generative AI models. By utilizing these procedures, we streamline healthcare and life sciences operations, assuring peak performance and efficiency in producing beneficial insights and breakthroughs for the sector.
Further Read: Is Your Organization Ready for Generative AI
Wrapping Up
Generative AI’s integration into healthcare and life sciences promises transformative advancements in medical research, diagnostics, and patient care. Use cases, from drug discovery to data augmentation for model training, demonstrate its potential to revolutionize these industries. Exciting opportunities for innovation lie at the intersection of technology and life sciences, encouraging continued exploration for the betterment of patient care.
In our podcast, Mr. Deepak Mittal, and Mr. Partha Anbil highlighted the significance of robust data systems and strategic alliances to unlock Generative AI’s true potential. At NextGen Invent, we are committed to being at the forefront of AI enabled solution development and empowering businesses in the healthcare and life sciences sector. Our team of experts is dedicated to overcoming challenges and harnessing the potential of Generative AI.
Contact us today to explore how our innovative AI solutions can revolutionize your organization and drive progress in healthcare and life sciences.
Stay In the Know
Get Latest updates and industry insights every month.