How is Predictive Analytics in Healthcare Revolutionizing It?
Custom AI mhealth app development is transforming the way healthcare companies function, from controlling population health to customizing treatment regimens for chronic illnesses. It’s about harnessing big data to make quicker, more informed decisions that lower healthcare costs and enhance patient outcomes.
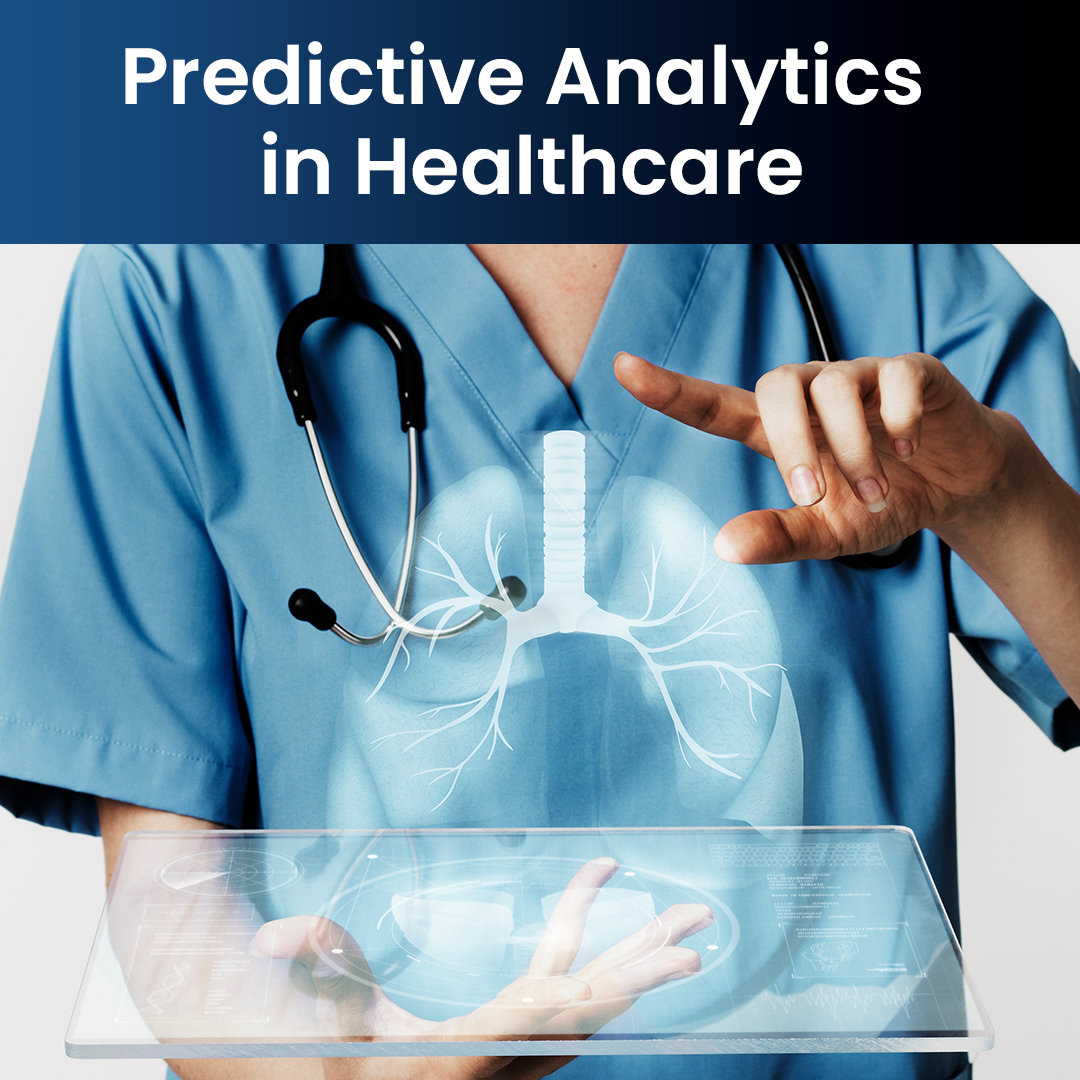
Overcome Data Silos Through Seamless AI Integration in Your mHealth App Development Journey
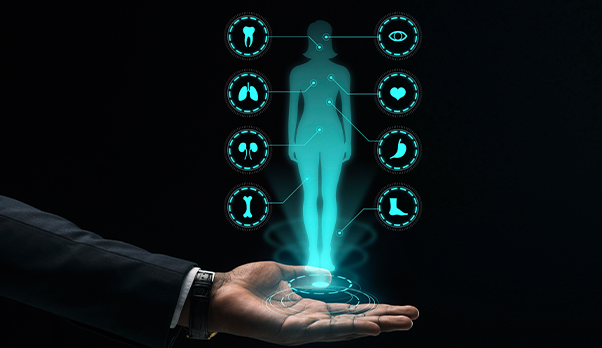
“Health care has a long track record of evidence-based clinical practice and ethical standards in research. However, the extension of this into new technologies such as the use of predictive analytics, the algorithms behind them, and the point where a human mental process should replace a machine process is not regulated or controlled by industry standards.”– Deloitte
Overview of Predictive Analytics in Healthcare
Predictive analytics in healthcare makes use of technology to create more effective, economical systems and enhance the health of individuals and the community as a whole. To analyze even the most complicated information, the approach fully utilizes cutting-edge data analysis tools, including deep learning, machine learning, big data, and artificial intelligence.
- It has the potential to revolutionize every aspect of the sector, including global health maps, hospital administration, and patient care.
- Stated differently, it signifies a shift from a treatment-based, reactive strategy to one that is more preventive.
What does that signify? In the medical field, prompt interventions and prevention can make the difference between life and death. The analytics algorithms push that line farther away using data gathered from multiple sources. These sources include genetics, family history, wearable medical technology, and electronic health records. On a larger scale, socioeconomic information and ethnic predispositions are also included in the models. When combined, they produce a network of suggestive crosspoints that are prepared for additional interpretations.
Predictive Modeling in Healthcare
Healthcare predictive analytics software analyzes current and historical data using algorithms and statistical methods. Medical records, demographic data, and patient histories all aid in spotting trends and connections that could otherwise go unnoticed. In addition to predicting the results of specific patients, they can also predict more general health trends, such as the potential emergence of infectious diseases or the future need for medical services. By taking a global view, predictive analytics in healthcare can impact global health management and large-scale health trends in addition to providing benefits for individuals.
- Predictive analytics can help guide public health planning and policy by examining health trends and patterns. This information can be used by governments and global health organizations to plan immunization campaigns, distribute funds, and carry out preventative health initiatives.
- Health operations can track and forecast illness trends globally with predictive analytics in healthcare projects. This is essential for predicting and controlling infectious disease outbreaks, like pandemics like COVID-19 or flu epidemics.
- International healthcare collaboration is fostered by the use of predictive analytics in healthcare projects. This helps healthcare systems around the world by facilitating coordinated global responses to health emergencies.
Why is Predictive Analytics Important for Healthcare?
Clinical treatment and operational effectiveness both benefit from predictive analytics. Healthcare professionals can deliver better treatment regimens that keep patients healthy for longer and at a lesser cost when they can identify health risks with accuracy. Better risk estimations for cardiovascular disease are produced using predictive analytics that uses social and environmental patient data, according to recent studies. Clinicians can use these findings to keep patients who are at risk from experiencing worsening symptoms that might require hospitalization.
From an operational standpoint, predictive analytics assists healthcare providers in addressing workforce shortages and optimizing capacity management. Up to 94% of registered nurses say there is a moderate to severe nursing shortage in their area, and 80% predict that the shortfall will worsen over the next five years. To better respond to viral outbreaks and stop the spread of illnesses, public health officials also require predictive analytics. Predictive analytics, for instance, has previously been used to forecast the spread of influenza at the state and local levels.
Enhance Operational Efficiency by Automating Workflows Using Our AI-Driven mHealth App Solutions
Predictive Analytics in Healthcare: Top 10 Use Cases
Analytics can have a huge impact on the healthcare sector as technology advances. Data can be used by AI and machine learning techniques to detect illnesses, choose the best course of action for each patient’s unique use case, and much more. The following are the main use cases that predictive analytics can help healthcare organizations:
1. Chronic Disease Management
In addition to being the primary causes of the $3.5 trillion in yearly health expenses in the US, chronic diseases are the leading causes of death and disability in the nation. 75% of healthcare expenditures are related to five chronic diseases: renal disease, diabetes, obesity, cardiovascular disease, and cancer.
The ability of medical practitioners to both prevent and regulate the development of chronic diseases is essential to their management. However, managing and preventing chronic illnesses is a difficult undertaking. To give patients more effective therapies at a lower cost, predictive analytics can enable healthcare practitioners to make prompt, fact-based decisions.
2. Improved Hospital Administration & Operational Efficiency
By avoiding downtime brought on by scheduling conflicts or delays in processing claims, predictive analytics in healthcare can help the administrative side. Workflow procedures can be optimized in this way, becoming more streamlined and effective. Appointments can be scheduled at maximum capacity with the aid of predictive analytics, which can assist in forecasting patient flow. It also makes it possible for patients, insurance providers, and hospitals to work together more effectively to process claims.
Additionally, healthcare workers can operate in a stress-free atmosphere and concentrate on delivering better and more effective customer service by using predictive analytics to automate time-consuming procedures. To sum up, predictive analytics improves operational efficiency and helps healthcare providers retain patients in-network.
3. Early Intervention for Disease Progression & Comorbidities
The life-saving benefits of early intervention in progressive diseases and concomitant disorders have long been recognized by medical professionals. However, without strong enough analytical capabilities to handle data sets big enough to smooth out anomalies, early intervention frequently remains a well-meaning conjecture.
Predictive analytical techniques have recently been used by healthcare organizations to analyze vast amounts of case histories about progressive diseases. Promising outcomes in risk assessment for cardiovascular disease and diabetic complications have already been obtained using this application. Since fewer patients develop life-threatening disorders and caregivers can focus on other needs, increasing the overall effectiveness of treatment for common chronic diseases can have a positive long-term impact on healthcare organizations.
4. Preventing Readmission
Readmissions to hospitals come at a hefty price. Readmissions cost the US healthcare system about $52.4 billion annually. Medicare’s Hospital Readmission Reduction Program, which offers financial incentives to prevent readmission, also imposes severe fines on hospitals. 82% of participating hospitals were hit with this kind of fine.
Predictive analytics in healthcare can identify patients who exhibit characteristics that suggest a high likelihood of readmission, allowing physicians to customize discharge procedures and devote more resources to follow-ups to avoid a rapid turnaround.
Reshaping Patient Engagement and Remote Patient Monitoring
5. Predicting Suicide Attempts
Every year, 14 Americans out of 100,000 die by suicide, making it the tenth most common cause of death in the US. To predict the possibility of future attempts, healthcare predictive analytics algorithms can take into account a patient’s medical history, past attempts, and social and economic circumstances. A research team at the Vanderbilt University Medical Center (VUMC) created a predictive analytics model that predicts the probability of suicide attempts by specific patients based on their electronic medical information. For eleven months, the instrument was evaluated at VUMC. The algorithm predicted the likelihood that patients would return for treatment for attempted suicide while doctors were treating patients.
Patients were divided into eight categories by this healthcare predictive analytics platform according to their predicted risk variables. The highest-risk category was responsible for more than 33 percent of all suicide attempts, according to the VUMC research team. The panel concluded that individuals assigned to high-risk groups ought to have their suicidal tendencies evaluated. In another instance, researchers used predictive analytics in the medical field to identify suicidal thoughts and attempts in adult South Koreans. When examining variables like income, self-esteem, and depressive symptoms, they obtained great accuracy.
6. Forecasting Appointment No-Shows
Appointment no-shows cost the US healthcare system over $150 billion annually, not including the administrative strain they cause. Each missed appointment costs an average of $200 for individual practitioners. By predicting which patients miss appointments without warning, AI demand forecasting software solution can increase cost-effectiveness for both private clinics and hospitals. They can thereby raise provider satisfaction and stop revenue loss.
Researchers partnered with Chile’s Doctor Luis Calvo Mackenna Hospital, a pediatric institution that reported a high 29% no-show rate. The researchers processed patient data, including demographics and social circumstances, using healthcare predictive analytics and machine learning to identify patients who are likely to miss appointments and remind them by phone. Throughout the eight-week trial, the researchers were able to decrease the number of no-shows by 10.3%.
7. Enhance Cybersecurity
The HIPAA Healthcare Data Breach Report states that cyberattack are common in the medical field. According to the research, hacking was the most frequent reason for the more than 82.6 million compromised medical records that occurred between January and October 2023.
Cybersecurity predictive analytics has the potential to improve the state of healthcare. Real-time risk scores for various online transactions can be computed by healthcare companies using predictive analytics and artificial intelligence solutions for the medical industry. Based on these scores, events can be responded to.
8. Preventing Patient Deterioration in ICUs and General Hospitals
Physicians and nurses must act fast to identify any deterioration in a patient’s health in both conventional hospital wards and intensive care units. When quick action might mean the difference between life and death, this is particularly true. Even before the COVID-19 epidemic, this was a problem. An increasing older population, complex surgical procedures, and a shortage of critical care specialists have already put a strain on intensive care units in several nations, including our own. The healthcare industry is in dire need of technical assistance to make prompt, well-informed judgments as the epidemic continues to aggravate the situation. It can identify patients who are likely to need assistance within the next hour by continuously monitoring their vital signs. This makes it possible for caretakers to intervene as soon as health starts to deteriorate. Predictive analytics is used in healthcare to determine the likelihood that a patient will die or require readmission within two days of leaving the intensive care unit. With this information, caregivers can make well-informed decisions about patient release.
Furthermore, in general, wards, where these indicators can go undiscovered for a long time, predictive analytics aid in identifying the first indications that a patient is beginning to perform poorly. According to a Philips report, automated early warning systems enable Rapid Response Teams to respond promptly, leading to a significant decrease in adverse events by 35% and hospital heart attacks by 86%.
9. Predicting Patient Preference
An essential component of delivering high-quality healthcare is being aware of patient preferences. Predictive analytics in healthcare can reveal information about treatment preferences and patient decisions. By empowering doctors to take a patient-centric approach, this method improves patient satisfaction and treatment outcomes. Predictive analytics, for example, can determine which doctor best suits a patient’s needs by looking at their previous choices.
Additionally, predictive analytics can forecast a patient’s propensity to follow a certain treatment plan by examining lifestyle information and medical history. For instance, some patients prefer modern treatment, while others are more inclined to adhere to an Ayurvedic regimen.
10. Handling Insurance Claims
The use of predictive analytics in health insurance is rapidly expanding. To accurately predict a patient’s health risks and determine insurance premiums, insurance firms are taking advantage of this technology. Additionally, it gives them the ability to design personalized coverage plans that cater to the needs of every patient. In the healthcare industry, advanced analytics has also been instrumental in revolutionizing the claim reimbursement procedure. Hospitals can benefit from faster insurance reimbursements by having better financial flow. It can assist companies in keeping up with operating costs, providing high-quality patient care, and streamlining administrative procedures. This can improve the institution’s overall stability and financial health.
Healthcare companies can review their applications before submission by using predictive analysis. The program can forecast whether a reimbursement claim will be successful or not. To assist with its billing procedures, the Cleveland Clinic uses a natural language processing system driven by artificial intelligence to extract information from unstructured data. Their invoicing procedure is now automated, resulting in zero errors and speedy refunds.
Boost Care Coordination with Custom AI mHealth Apps Offering Real-Time Data Insights and Alerts
The Future of Predictive Analytics in Healthcare
- Integrating Artificial Intelligence: Predictive modeling in healthcare could advance with the use of artificial intelligence and predictive analytics. Large volumes of data may be processed and analyzed more quickly by AI-driven predictive analytics models, which can then produce ever more precise forecasts and useful insights.
- Leveraging Real-Time Data: Healthcare professionals can collect patient data in real-time by connecting wearable technology with other IoT technologies. This real-time information will strengthen the accuracy of predictive models, enabling more rapid interventions and enhanced patient care.
- Expanding the Use of Machine Learning Models: The use of deep learning and machine learning techniques is essential to the development of predictive analytics in the medical field. Over time, these methods increase their forecast accuracy by continuously learning from new data. Healthcare professionals will be able to provide more individualized care and keep ahead of new health trends by utilizing machine learning in predictive analytics.
Collaborate with NextGen Invent to Enhance Your Healthcare Development Services with Predictive Analytics
Unquestionably, the healthcare sector is bursting at the seams with data—patient records, medical imaging, laboratory testing, research studies, and pharmaceutical trials—and the amount of information produced is massive. This information could improve resource use, support patient care, and address global health concerns. Unlock the potential of data across health systems and datasets with our robust custom AI mhealth app development services.
Our team’s proficiency in complex predictive analytics gives healthcare businesses a contextualized, real-time perspective of their data, enabling healthcare workers to make more informed decisions and improve patient outcomes.
Frequently Asked Questions About Predictive Analytics in Healthcare
Related Blogs
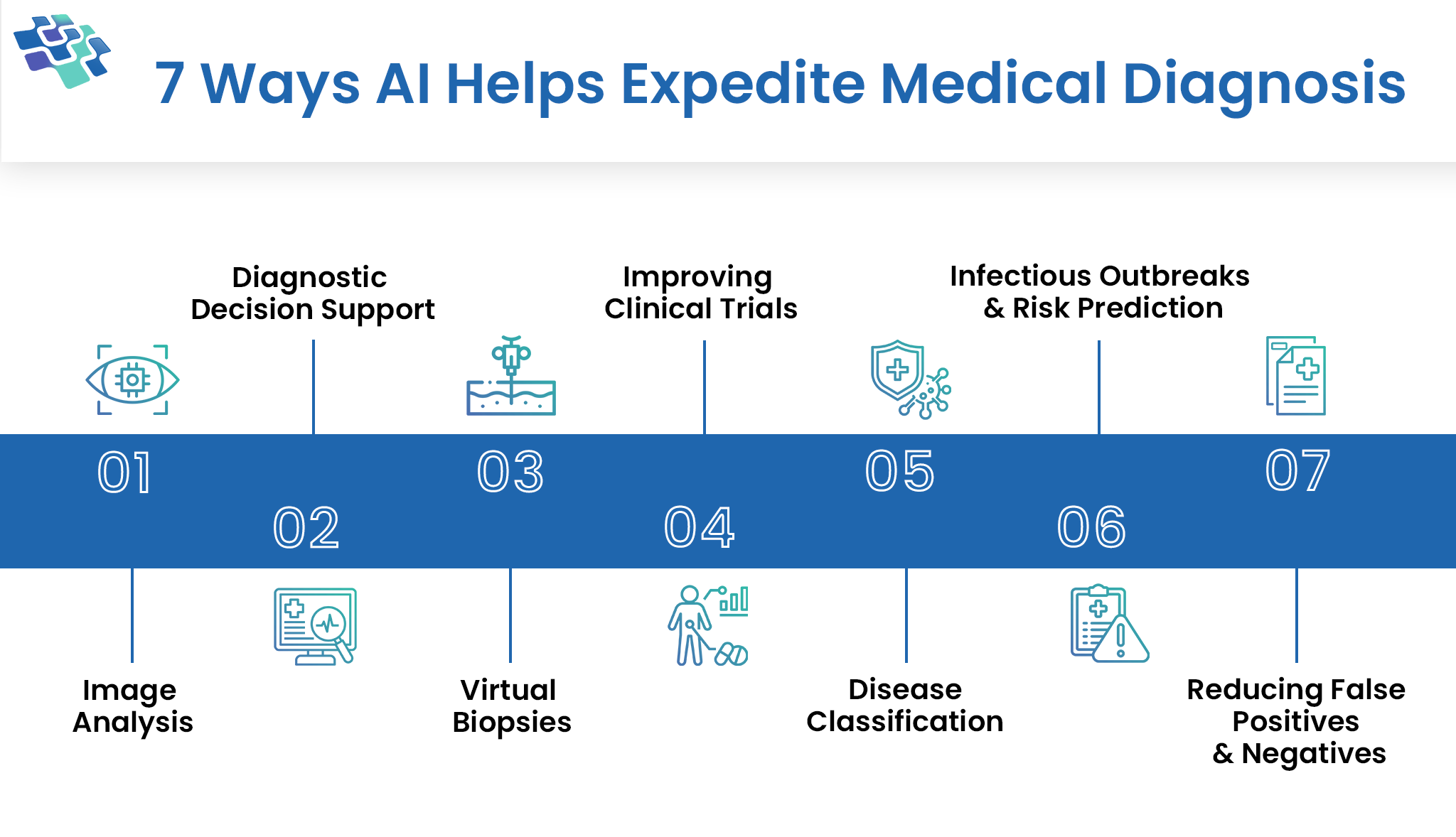
How Does AI in Medical Diagnosis Expedite It?
One of the most noteworthy developments in the healthcare industry in recent years has been the use of AI in medical diagnosis and treatment choices.
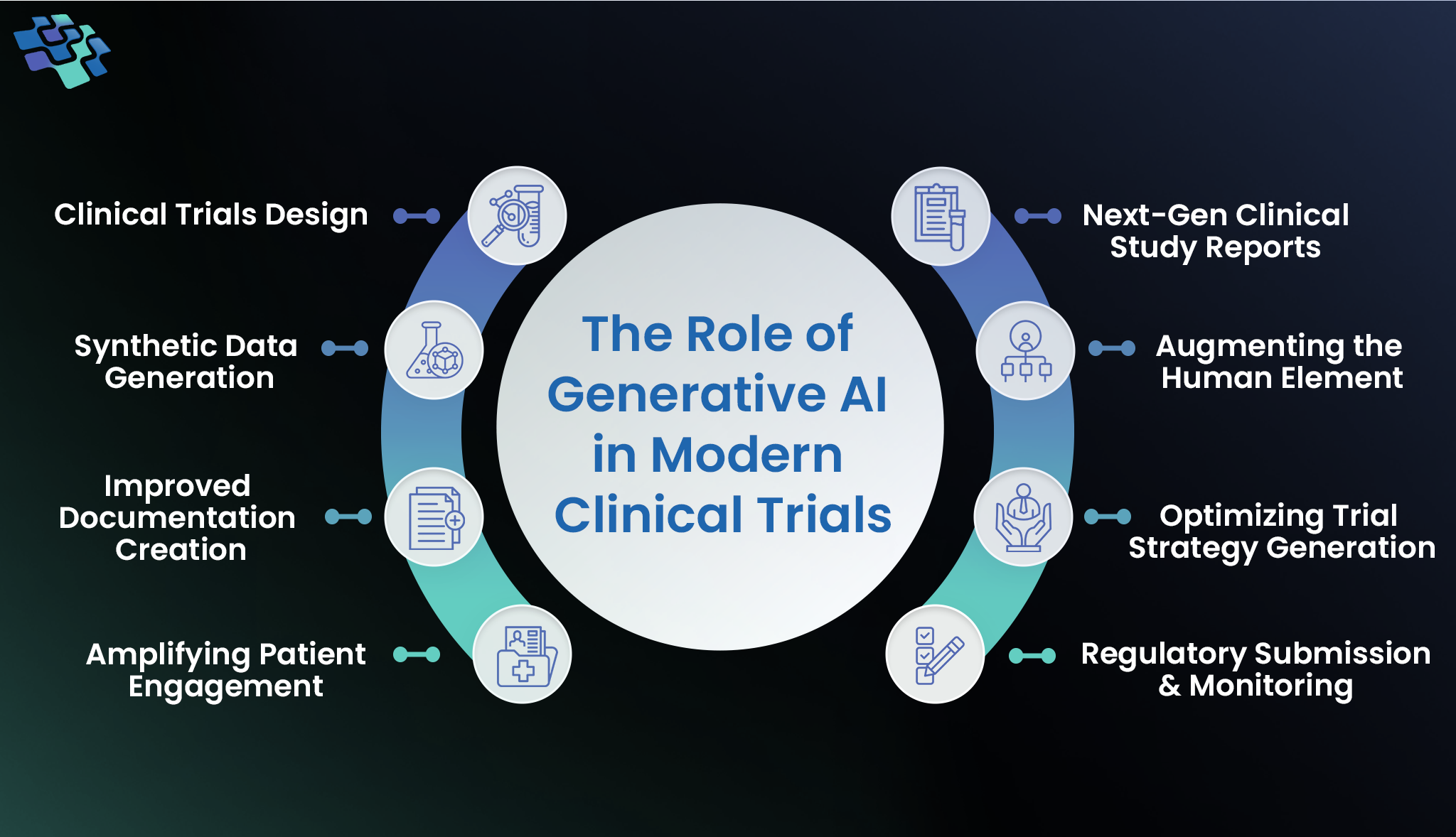
The Advancing Role of Generative AI in Clinical Trials
Generative AI in clinical trials has potential because these trials should be cost-effective, versatile, and adaptable to meet the varied objectives of different stakeholder groups.
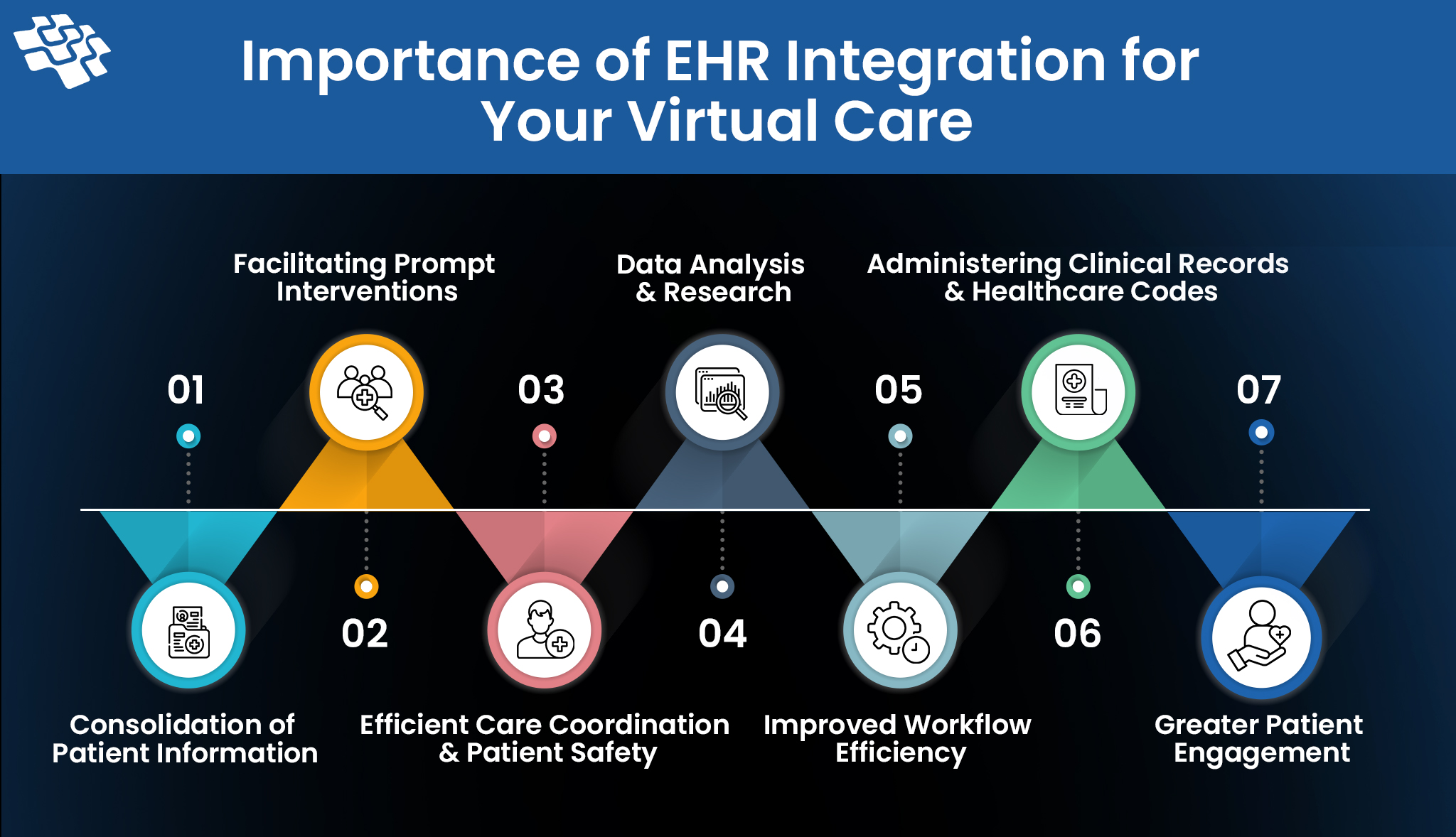
Importance of EHR Integration for Your Virtual Care
Did you know that 60% of patients concur that virtual care is more convenient than receiving therapy in person? Virtual care is clearly here to stay, especially when you consider...
Stay In the Know
Get Latest updates and industry insights every month.