Use Cases of AI in Demand Forecasting Across Supply Chain Verticals
Realistic sales targets improve the effectiveness of supply management execution, while accurate demand forecasting lowers the danger of overstocking or underproduction. Demand forecasting is difficult for businesses because of their wide range of products and large customer base. As a result, they need AI demand forecasting software solutions that can accurately estimate demand.
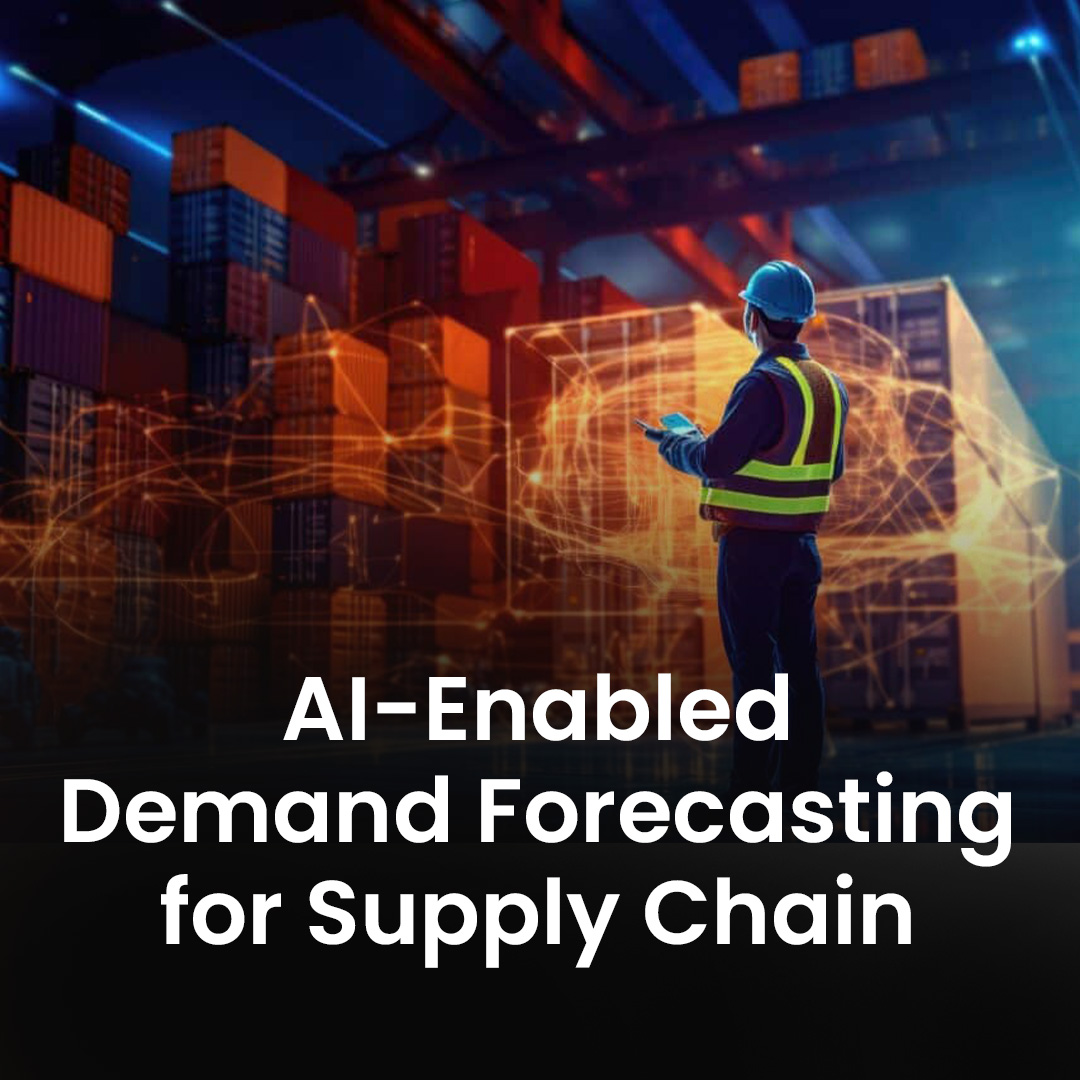
Battling Supply Chain Disruptions? Our Advanced AI Solutions Build Resilience and Flexibility into Your Operations
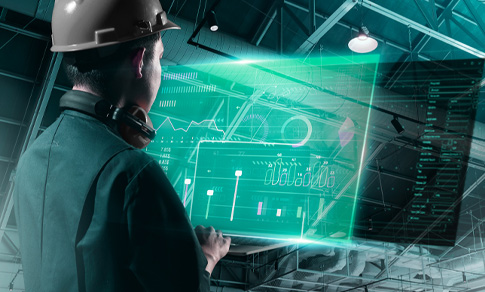
“Applying AI-driven forecasting to supply chain management, for example, can reduce errors by between 20 and 50%—and translate into a reduction in lost sales and product unavailability of up to 65%. Continuing the virtuous circle, warehousing costs can fall by 5 to 10%, and administration costs by 25 to 40%.”– Mckinsey
What is AI-Enabled Demand Forecasting for Supply Chain?
The term “demand-driven” refers to the conventional method of inventory forecasting. It is predicated on client order data, which is subsequently utilized to forecast future inventory requirements. This method of inventory management works well, but it might cause issues if demand is excessive or unpredictable. For most businesses, this results in overstocking and understocking, which is expensive.
By optimizing the forecasting process by considering past sales data, current trends, and social media buzz, AI-enabled demand forecasting improves logistics. This data is enhanced by AI, which also extracts valuable information to forecast resource needs in advance. By applying AI in demand forecasting, logistics operators can make quantitative and precise demand estimates across the supply chain.
Problems with Traditional Supply Chain Forecasting Methods
In today’s changing market, traditional supply chain forecasting techniques frequently need to catch up. The lack of real-time data analysis is one of their main drawbacks, which makes it difficult for them to react swiftly to unforeseen demand patterns or abrupt changes in the market. Forecasts and actual demand can diverge significantly because of this rigidity.
- Numerous case studies draw attention to these flaws. A major retailer might, for example, stock up on winter apparel based on past sales statistics, only to have a hot winter. This would result in excess inventory and steep discounts to get rid of the product. These incidents highlight how the limitations of conventional forecasting techniques affect company operations and profitability, resulting in missed opportunities and resource waste.
The inability of conventional forecasting techniques to adjust to changes in the market is one of their disadvantages. They frequently use oversimplified models that fall short of capturing the intricacy of contemporary supply chains, neglecting elements that might significantly impact demand, such as social trends, economic fluctuations, and geopolitical events. The shortcomings of these approaches have practical repercussions in addition to being theoretical.
Businesses that use antiquated forecasting methods risk falling behind their nimbler competitors who use cutting-edge technologies. There may be serious financial repercussions for businesses, including decreased sales, higher operating expenses, and strained client relationships.
How AI in Demand Forecasting Differ from Traditional Forecasting Methods?
Forecasts produced by traditional forecasting techniques are frequently less accurate since they are unable to account for contextual elements like consumer behavior, market trends, and outside influences. These approaches assume that past trends will persist in the future unaltered. Their applicability can be limited by their inability to handle complex patterns or enormous volumes of data.
AI forecasting, on the other hand, makes use of advanced algorithms for machine learning that can swiftly evaluate enormous volumes of data and spot complex patterns and trends. AI models quickly update projections to account for unforeseen events, such as unexpected product spikes or operational outages. To precisely estimate future demand, a standard demand forecasting solution makes use of a variety of data types. Among these data are:
- Historical sales data,
- Economic conditions,
- Real-time data, such as website traffic and social media engagement,
- Competitor activity.
Benefits of AI in Demand Forecasting
Numerous benefits of AI in demand forecasting have already been covered. The main benefits that AI powered demand forecasting can offer any manufacturing business are as follows:
- Better Markdown/Discount Optimization: Businesses frequently experience cash-in-stock situations, in which merchandise is unsold for longer than anticipated. This frequently results in increased anticipated inventory expenses as well as the chance of products losing value and going out of style. The products are offered for sale at a reduced price in this case. Demand forecasting can reduce the likelihood of this happening.
- Overall Effectiveness: Instead of trying to use demand forecasting to change personnel and inventory levels, teams can focus on strategic issues.
- Higher Customer Contentment: Customer satisfaction decreases when products are “out of stock,” but it increases when they are consistently available. As a result, brand perception and customer loyalty increase.
Facing Demand Uncertainty? Let Our AI Software Development Services Enhance Predictive Accuracy and Drive Better Planning
Use Cases of AI in Demand Forecasting Across All Supply Chain Verticals
By utilizing complex Large Language Models and linking them with an organization’s vast data sets, artificial intelligence revolutionizes conventional methods for demand forecasting. This innovative method improves data analysis and produces profound insights, which enable accurate forecasting and assist companies in managing supply chains, optimizing inventories, and coordinating production with market trends.
1. Historical Data Analysis & Predictive Analytics
AI can find patterns and trends in past sales data by accounting for variables like holidays, promotions, and seasonality. Businesses can better forecast future demand by using this data to understand historical consumer behavior. Real-time data from a variety of sources, such as social media, online transactions, and point-of-sale systems, can be analyzed by AI algorithms.
Supply chain managers can improve inventory levels and prevent stockouts or overstock problems by using this data to forecast future demand better. By spotting patterns and trends that conventional approaches can overlook, machine learning-based inventory demand forecasting improves inventory management and increases inventory planning accuracy.
2. Warehouse Space Optimization & Production Planning
Optimizing space usage in response to anticipated shifts in product demand is the goal of a warehouse manager. Ai based demand forecasting methods examine market trends, order history, and SKU-level data. By using this data to forecast which products will see a spike in demand, the warehouse can better manage its inventory by modifying storage arrangements and allocating space appropriately.
A maker of electronics must coordinate production with fluctuating demands for final products and depend on several suppliers for components. To precisely predict component demand, AI-driven supply forecasting algorithms examine production lead times, supplier performance metrics, and historical order data. This makes it possible for the manufacturer to work with suppliers, optimize production scheduling, and avoid shortages or surplus inventory at various supply chain points.
3. Customer Behavior Analysis & Satisfaction
AI uses data from a variety of sources, such as online platforms and customer support exchanges, to analyze client behavior. Manufacturers can obtain important insights by using predictive models, which carefully identify patterns and trends in consumer preferences. By deliberately adjusting production quantities and improving product offers, supply chain managers can ensure a proactive reaction to expected shifts in customer demand and promote a more flexible and customer-focused manufacturing approach.
Precise demand forecasting ensures that goods will be accessible when consumers desire them. Because companies can better satisfy customer expectations and prevent situations when popular products are out of supply, this improves customer satisfaction.
The Transformative Impact of AI in Supply Chain
4. Supplier Collaboration & Production Planning
AI enhances supplier collaboration and production planning by enabling real-time data sharing and communication, fostering stronger partnerships across the supply chain. Through predictive analytics, AI analyzes historical data, market trends, and demand fluctuations to forecast material needs accurately. This allows suppliers to adjust production schedules proactively, ensuring timely inventory replenishment and minimizing stockouts or excess inventory. AI-driven insights also support demand alignment across the supply chain, allowing manufacturers to optimize production rates based on predicted needs.
Additionally, AI-powered systems enhance visibility into supplier performance, helping identify potential risks and improve lead times. By automating these processes, companies can build more resilient, agile, and responsive supply chains, boosting overall efficiency. This collaborative approach to production planning not only reduces costs but also enhances product availability and responsiveness to market changes.
5. Real-Time Insights & Personalized Demand Prediction
AI in demand forecasting makes it possible to analyze a variety of data sources in real-time, such as emails, market trends, social media platforms, and economic indicators. Businesses can react swiftly to shifting market conditions because of the real-time insights this ongoing study provides.
Demand planning with AI uses real-time interactions and historical data to customize projections to each customer’s preferences. It facilitates better client segmentation tactics and improves product recommendations to satisfy customer wants. Demand forecasting with AI is not merely a technical advancement; it is a calculated step that can revolutionize company operations and increase their competitiveness, resilience, and efficiency.
6. Cost Reduction & Increased Profitability
Businesses can drastically save expenses related to overstocking and understocking by reducing forecasting errors.
- For example, it can assist in reducing administration costs by 25–40% and storage expenses by 5–10%, resulting in significant savings.
Increased profitability is the result of the combined advantages of AI-based demand forecasting, which include cost savings, operational effectiveness, customer satisfaction, etc. By reducing wasteful spending and optimizing return on investment, businesses can open new growth and innovation opportunities.
Need Real-Time Insights? Our AI Software Services Provide Actionable Data to Streamline Your Supply Chain Processes
The Future Trends in AI-Powered Demand Forecasting and Supply Chain Planning
Exciting opportunities abound for demand forecasting driven by AI in the future:
- Advancements in Machine Learning Algorithms: Demand forecasting models will get even more advanced in their capacity to manage intricate and non-linear interactions between variables as machine learning algorithms advance.
- Future Technologies in Supply Chain Management: Supply chain visibility and forecasting accuracy will be further improved by combining AI with other cutting-edge technologies like blockchain and the Internet of Things (IoT).
- The Role of Big Data Analytics: More potent AI models will be fueled by the constantly expanding amount of data available, resulting in increasingly more predictive powers.
Demand forecasting driven by AI is not only revolutionizing supply chain planning, but it is also turning into a strategic requirement for companies looking to maintain their competitiveness in a market that is changing quickly. Businesses that use AI-driven solutions will be well-positioned to streamline operations, cut expenses, and react more quickly to market needs as the worldwide supply chain management market is expected to grow to USD 63.77 billion by 2032.
Conclusion
Demand forecasting with AI has the potential to completely transform how businesses anticipate and react to market developments. Businesses can attain previously unheard-of levels of accuracy and efficiency in their forecasting operations by utilizing complex algorithms and real-time data integration. By facilitating proactive decision-making, cutting expenses, and improving customer satisfaction, it puts businesses in a position to remain competitive.
At NextGen Invent, our AI based demand forecasting and inventory management solutions leverage advanced AI and machine learning to drive accuracy, efficiency, and growth in your business. Our specialized Artificial Intelligence software development services ensure robust data preprocessing, adaptable and scalable models and seamless integration with your existing systems. We deliver tailored solutions designed to address unique challenges and unlock the full potential of AI for more precise forecasting and efficient inventory management.
Frequently Asked Questions About AI in Demand Forecasting Across Supply Chain Verticals
Related Blogs
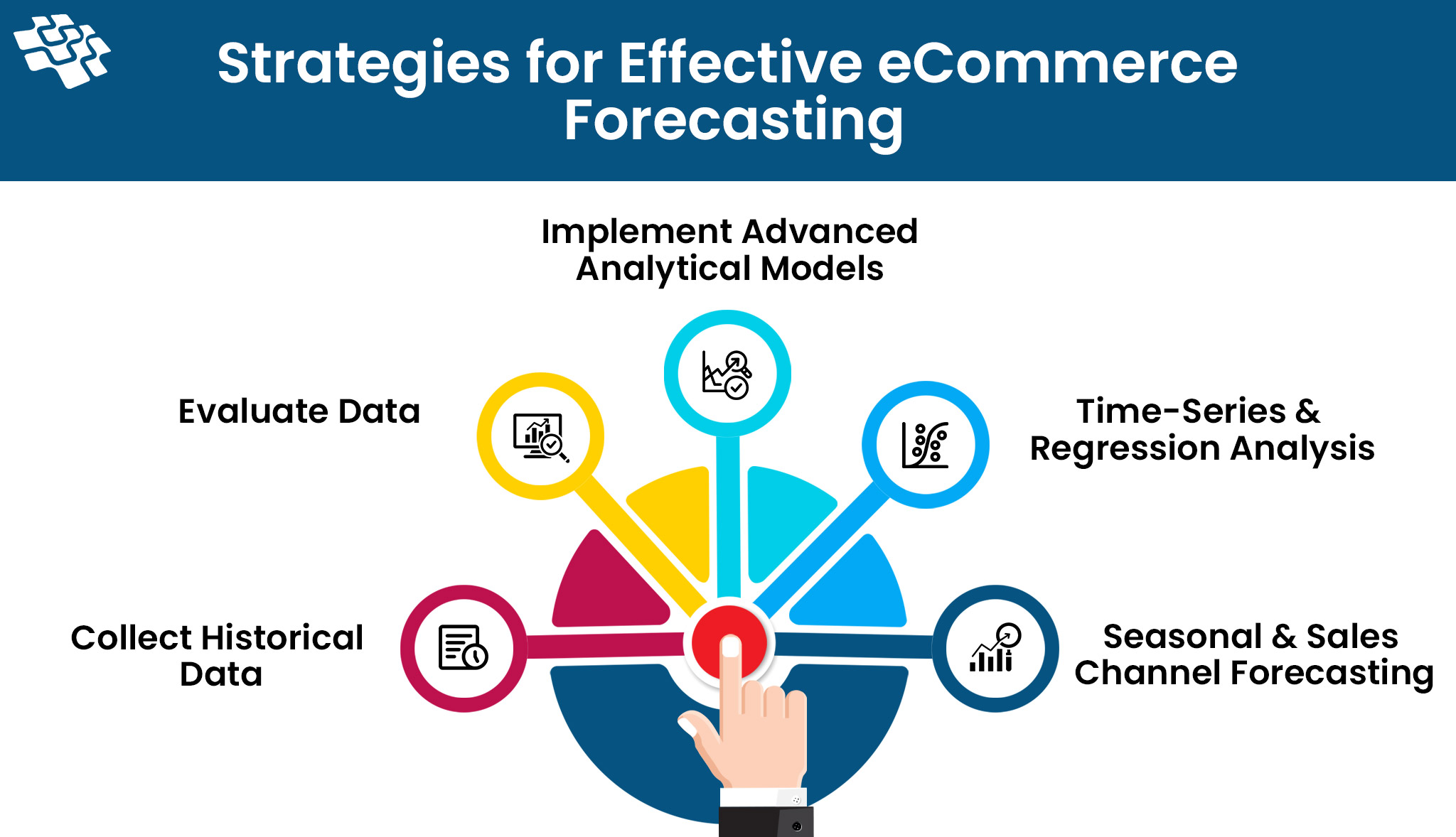
How to Win at Ecommerce Demand Forecasting in 2024?
People aren’t machines. They don’t always behave in the same ways, which can cause problems for an eCommerce business. For instance, a product that did well in sales the previous month may collect dust in a warehouse.
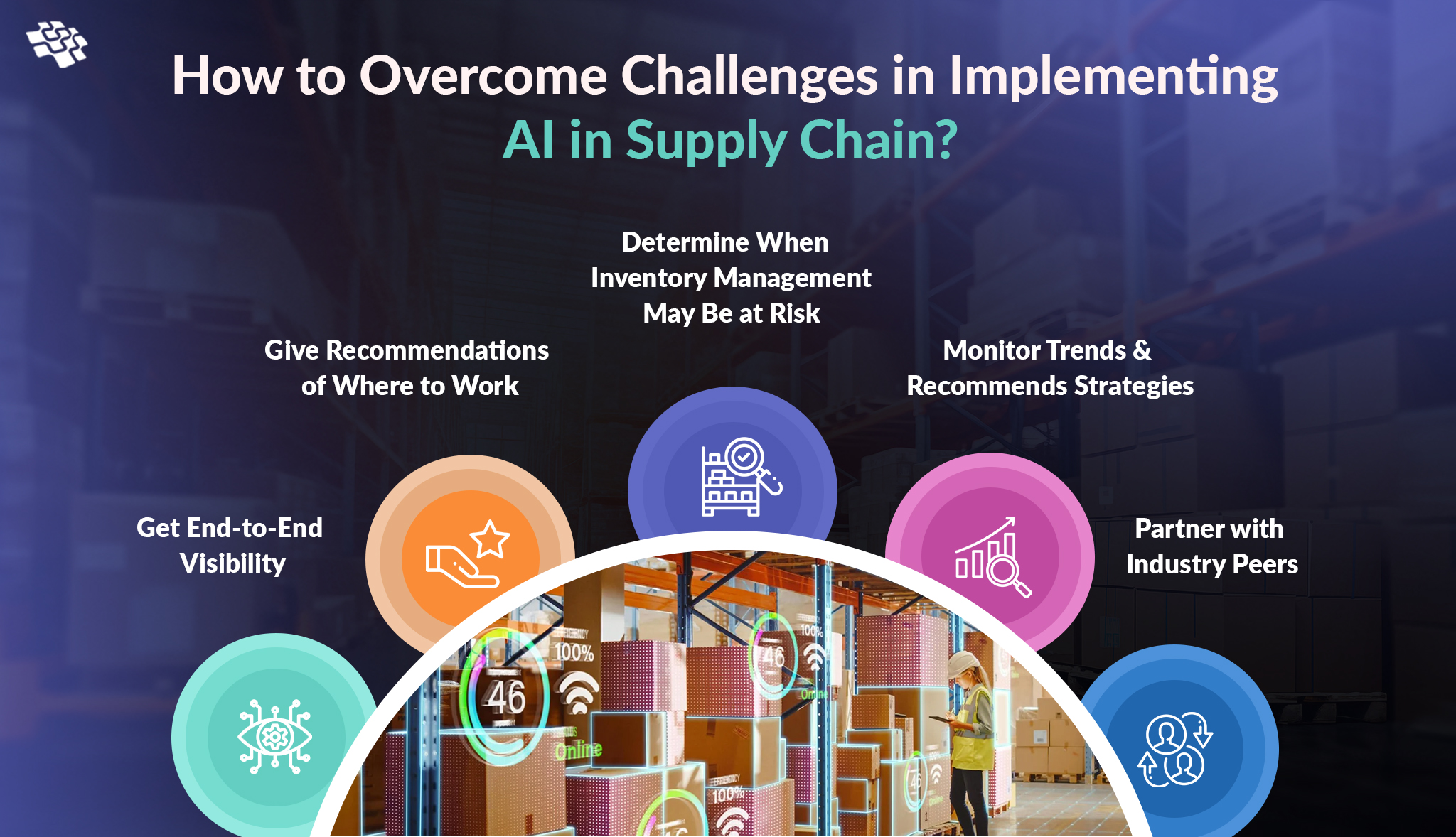
How to Overcome Challenges in Implementing AI in Supply Chain?
When it comes to the supply chain, AI technologies such as machine learning, deep learning, automation, and predictive analytics hold tremendous promise for transforming entire industries and organizations.
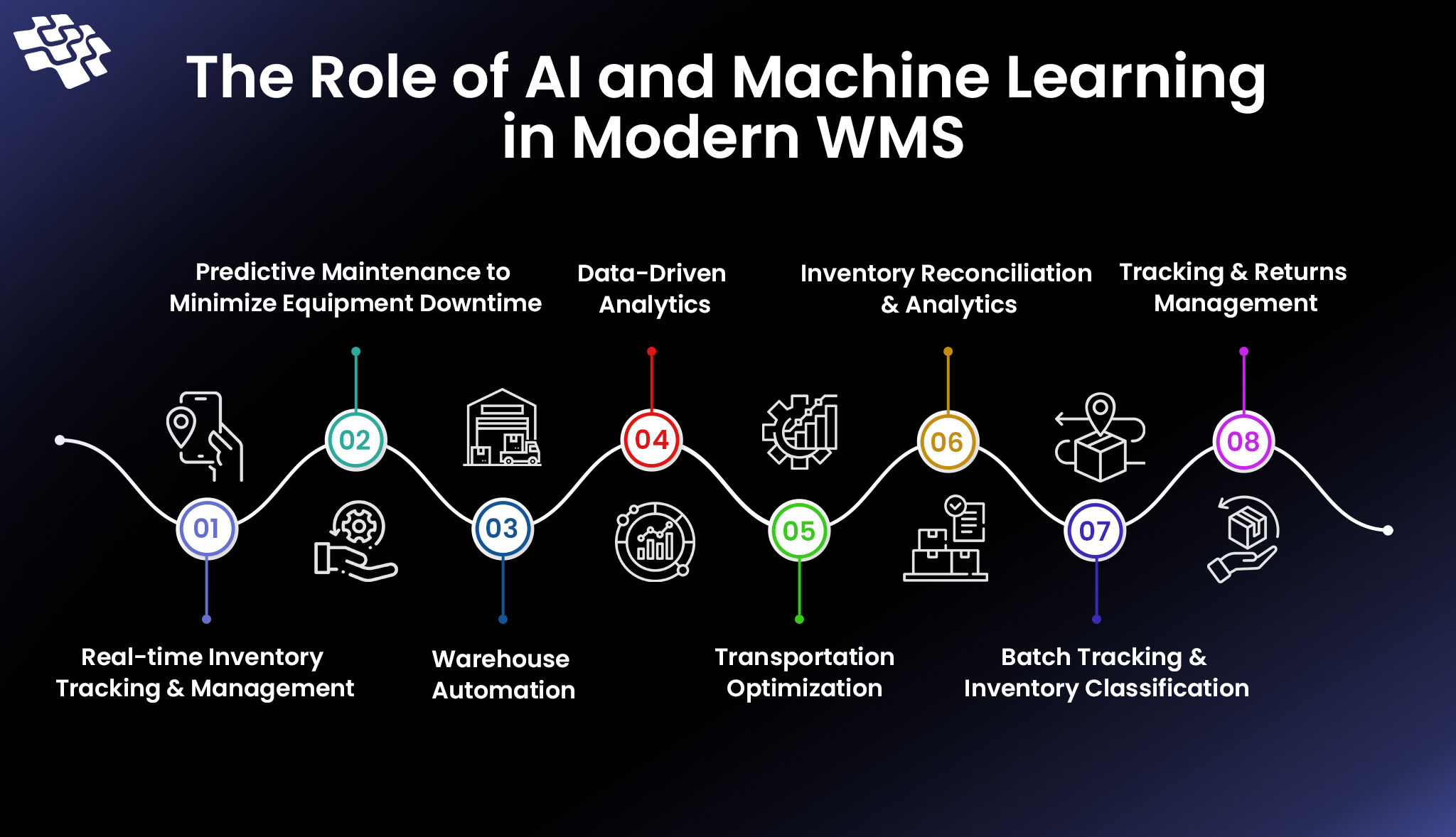
The Role of AI and Machine Learning in Warehouse Management
Warehouse management plays a significant role in supply chain operations. It ensures that goods are delivered, tracked, stored, and arranged as efficiently as possible.
Stay In the Know
Get Latest updates and industry insights every month.