The Advancing Role of Generative AI in Clinical Trials
In this blog, we examine how generative AI in clinical trials has the potential to transform clinical development and lead to more patient-centered, streamlined, and efficient methods.
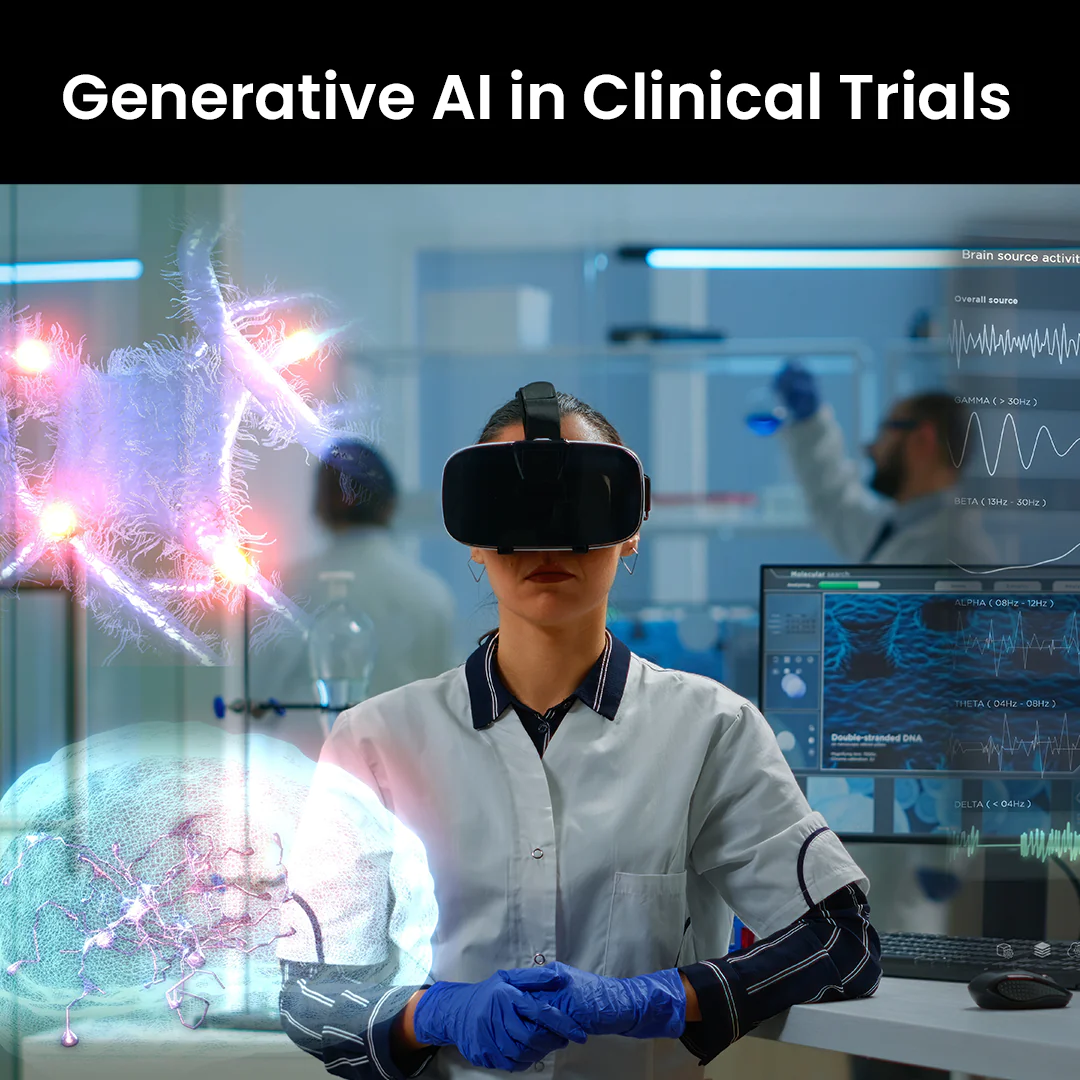
Need to Improve Patient Recruitment? Our AI-Enhanced Software Accelerates and Optimizes Patient Enrollment
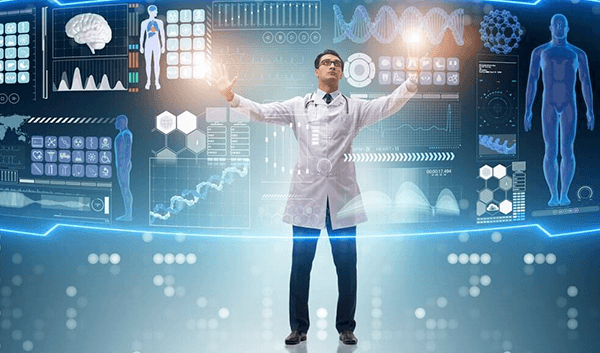
“Advanced technologies such as generative AI provide the potential to resolve many challenging areas of drug development which has several benefits for the pharmaceutical industries, patients, payers, and investigators. Gen AI-based study model helps to enhance and fasten the development of patient-centric models. This helps to decrease patient burden, boosts the likelihood of success, and augments overall efficacy.”– Market Research
Generative AI on Clinical Research: Crucial Impacts
Generative AI has quickly progressed from a new idea to a key player in changing clinical trial procedures. It plays many roles, including:
- Influencing protocol design,
- Patient group selection,
- Trial site selection,
- Regulatory compliance,
- Patient retention tactics,
- Clinical documentation management, and
- Adverse event monitoring.
By accelerating trial timeframes, increasing operational efficiency, and improving regulatory compliance, the integration of generative AI in clinical trials across all areas aims to solve the most important problems and provide more effective, economical, and patient-focused healthcare solutions.
The difficulties faced by researchers and clinical practitioners are substantial, given that clinical studies frequently run six to seven years and patient recruiting costs an average of $1.2 billion. However, by offering advanced tools and insights, generative AI shows promise.
Key Trends of Generative AI In Drug Discovery
- A McKinsey report claims that by forecasting the subsequent chemical structure, generative AI can find a potential treatment for a specific ailment. This can speed up the drug discovery process 2.5 times and shorten the testing cycle.
- As per the same report, generative AI can cut expenses and time by 20% while increasing the likelihood that a clinical trial will be successful by 10%.
- AI-enabled workflows can reduce the time needed to bring new compounds for testing by up to 40% and the preclinical stage by 30%, according to Statista.
Challenges in Clinical Trials
- Data Management: Large volumes of data are produced during clinical studies, which must be precisely gathered, handled, and examined. Another level of complexity is added by making sure that data integrity and regulatory standards are followed.
- Patient Recruitment: One major obstacle in clinical trials is finding qualified subjects. Over 80% of clinical trials are thought to miss their enrollment deadlines, which causes delays and higher expenses.
- Complex Trial Design: It is difficult to design clinical trials that are both operationally and scientifically sound. Choosing the best trial design, endpoints, and procedures is part of this.
Benefits of Gen AI in Clinical Trials
The promise of Gen AI in pharma includes numerous potential advantages for clinical trial analysis and pharmaceutical drug development, such as:
- Accelerated Timelines: Gen AI drastically cuts down on the time needed to start and carry out clinical studies by streamlining patient recruiting and trial design. New therapies can reach the market more quickly because of this acceleration.
- Improved Accuracy: The qualities of future drug candidates as well as the efficacy and safety of current medications could be more accurately predicted by scientist-led efforts employing Gen AI by learning from vast databases of previous data.
- Improved Success Rates: Results from trials using generative AI enhancements are more likely to be legitimate and dependable. This lowers the chance of expensive trial failures and raises the possibility of market success and regulatory approval.
- New Insights: New ideas and insights can be produced by Gen AI’s capacity to connect disparate and large data sources. This can assist researchers in creating more potent medications and creating clinical trials with a higher chance of success.
Early Diagnostics Using Clinical and Molecular Data
The Role of Generative AI in Clinical Trials
Even though 90% of clinical studies fail, the process of creating a novel drug can take up to 12 years and cost an estimated $2.3 billion. Poor patient cohort selection, recruitment strategies, and inadequate infrastructure are frequently the causes of this. Clinical trials can be completed more quickly and efficiently with the aid of generative AI, which may also boost productivity in several other domains.
1. Clinical Trials Design
Scientists often spend hundreds of hours manually gathering and extracting data from diverse sources like patients, scientific journals, and trial paperwork. This time-consuming process is prone to errors and inconsistencies. However, by leveraging Generative AI, the efficiency of handling vast amounts of structured and unstructured data can be significantly improved. Gen AI automates data processing, reducing manual intervention and accelerating the initial evaluation of drug targets by up to 30%.
It enhances accuracy, minimizes human error, and ensures consistency across data sets, streamlining the research process. This technology has the potential to revolutionize early-stage drug discovery and improve decision-making in pharmaceutical development.
2. Synthetic Data Generation
Generative AI plays a pivotal role in clinical trials through synthetic data generation, especially when real-world patient data is limited or when there is no control group in randomized controlled trials (RCTs). Synthetic datasets, created to closely mimic actual patient data, allow researchers to conduct simulations and test hypotheses without compromising patient privacy.
Gen AI can generate synthetic control arms, reducing the need for traditional control groups, which is becoming increasingly supported by regulatory bodies. This accelerates trial timelines, enhances flexibility, and improves efficiency in drug development. By enabling researchers to work with synthetic data, it can significantly expedite the time to market for new treatments while maintaining robust trial results.
3. Improved Documentation Creation
Generative AI in healthcare enables organizations to rapidly create the documentation needed to set up new test sites by utilizing pre-existing clinical report forms, site contractual agreements, and clinical trial procedures. A clinical research strategy (also known as a clinical study protocol or clinical trial procedure) can serve as another illustration. It takes a lot of time and work to prepare and is an essential document to consult when performing the study. It includes a thorough description of every facet of the clinical trial. This procedure can be accelerated by generative AI.
Generative AI can also be used to optimize trial consent form preparation. The form should be written in plain text to make it understandable to the intended audience and is typically based on a protocol document. The production of consent forms is a laborious but simple procedure, making it a strong candidate for generative AI automation.
4. Patient Engagement Amplifying
For clinical trial locations, attracting and keeping patients can be a big challenge. By enabling customization on a large scale, generative AI can aid in patient recruitment. Additionally, it can generate personalized outreach and find the ZIP codes and physicians of participants who might benefit from a novel therapy.
Generative AI’s conversational nature can serve as the first line of defense when it comes to responding to patient inquiries, exchanging pertinent data, and addressing issues that may lead patients to withdraw from clinical trials early. The expense of attrition can reach $20k per patient. Organizations have a strong motivation to look into every possibility of keeping clinical trial participants because of that expense.
5. Regulatory Submission & Monitoring
The last stage before regulatory review is submission writing, which should be completed as soon as possible to shorten launch timelines. It usually takes eight or more weeks to draft clinical trial reports.
By employing the underlying protocol, statistical analysis plan, and other figures to generate an “80% right” first draft in minutes rather than days, generative AI-based solutions can nearly halve this time and expedite regulatory submission by 40%.
6. Trial Strategy Generation Optimization
Gen AI’s ability to quickly produce trial plans is one of its most significant uses in clinical trials. It can digest information in a matter of seconds by evaluating copious amounts of data from many sources. This allows it to simulate various situations and highlight the most promising and successful strategies. When users enter parameters like indication, phase, treatment area, desired timescale, and cost thresholds, generative AI will automatically assess potential outcomes and suggest the best course of action, along with recommendations for nations, locations, and hiring schedules.
This process’s iterative nature is what makes it captivating. Until they are satisfied users can evaluate the draft strategy, offer remarks, and seek changes. Then, usually, in a matter of seconds or minutes, Gen AI will use that input to build a new strategy. The main factor contributing to Gen AI’s speed advantage is automation, which frees up a significant amount of time and resources that were previously used by labor-intensive human workers.
7. Human Element Augmenting
A crucial component of integrating Gen AI into clinical trials is intelligent human augmentation. Trial monitoring is used to demand specialists to decipher intricate dashboards and visualizations. Generative AI improves data comprehension and transparency by enabling even non-experts to ask inquiries in natural language and get clear replies. In addition to providing stakeholders with more insights and ad hoc query capabilities, this “human-in-the-loop” idea ensures appropriate checks and human oversight over the AI system.
Additionally, through individualized study designs and efficient patient recruitment, Gen AI promotes clinical trial efficiency and patient-centricity. Precision matching of participants to appropriate trials is made possible by these technologies’ ability to discover ideal patient populations or subgroups through data analysis, and vice versa. This speeds up hiring, cuts down on expenses and schedules, and improves accessibility and communication for a range of stakeholders.
8. Next-gen Clinical Study Reports
Analyzing clinical trial data and condensing it to show statistical significance while upholding safety and efficacy criteria takes a lot of work. When done by hand, it is also prone to error. Conventional AI has been using probability and prediction to speed up this data collection for years. However, generative AI can uncover novel relationships that traditional models and human researchers might not see right away. For instance, a crucial statistic like trial recruitment velocity might be extracted by conventional AI. But with generative AI, the model may both provide suggestions for improvement and explain why that number exists.
One of the main benefits of generative AI is that all this information is presented understandably. Research teams wouldn’t have to go through multiple pages of Excel-based data or do calculations by hand to determine how strategy adjustments would impact results. It would also be unnecessary for them to compare data with ad buys or social media initiatives. Instead, the program compiles all that data into a concise list of suggested actions.
Struggling with Manual Data Management? We Develop Software Solutions that Provides Real-Time Data Monitoring for More Informed Decision-Making
Future Prospects
Here is a brief overview of generative AI’s prospects in research and clinical trials.
- Clinical trial procedures, from design to data processing, could be significantly improved by ongoing advancements in AI technology. More complex and accurate uses of AI are being made possible by advancements in data integration methods, machine learning algorithms, and natural language processing. These developments make it easier to create trials that are more flexible and effective, improve patient recruitment tactics, and increase data analysis precision, all of which contribute to quicker and more trustworthy clinical research results.
- To encourage innovation while maintaining safety and effectiveness, organizations are modifying laws to make it easier to incorporate AI in clinical studies. Regulatory agencies are trying to develop frameworks that permit the safe and efficient application of AI as they become more aware of how it might revolutionize clinical research. This entails establishing standards for patient privacy, data openness, and AI model validation and procedures for authorizing AI-driven clinical studies and therapies.
- Given their potential to revolutionize clinical research and medication development, pharmaceutical companies are embracing AI techniques at an accelerating rate. To speed up the development of novel treatments, cut expenses, and expedite clinical trial procedures, major industry participants are investing in AI technologies. The demonstrated advantages of AI in increasing trial efficiency, improving patient outcomes, and facilitating more accurate and individualized treatment approaches are what is driving this broad adoption.
Embracing Generative AI: Final Considerations
Generative AI holds immense potential in transforming clinical and pharmaceutical research, offering new avenues to accelerate drug development, enhance patient outcomes, and streamline clinical trials. While significant challenges exist—such as ensuring data privacy, addressing ethical concerns, and overcoming regulatory challenges—the opportunities for advancement are vast. By automating data analysis, generating synthetic datasets, and improving predictive modeling, Generative AI can reduce costs and time-to-market, ultimately benefiting patients worldwide. However, success in implementing these technologies requires a well-planned strategy that carefully evaluates both the benefits and risks.
At NextGen Invent, we specialize in clinical decision support system software solutions that integrate AI-driven insights, helping healthcare organizations navigate these challenges effectively. Our generative AI software development in healthcare solutions enables faster, more accurate decision-making, ensuring that patient care is optimized with innovative technology. Contact us today to stay ahead in the AI revolution and drive innovation in clinical research.
Frequently Asked Questions About Generative AI in Clinical Trials
Related Blogs
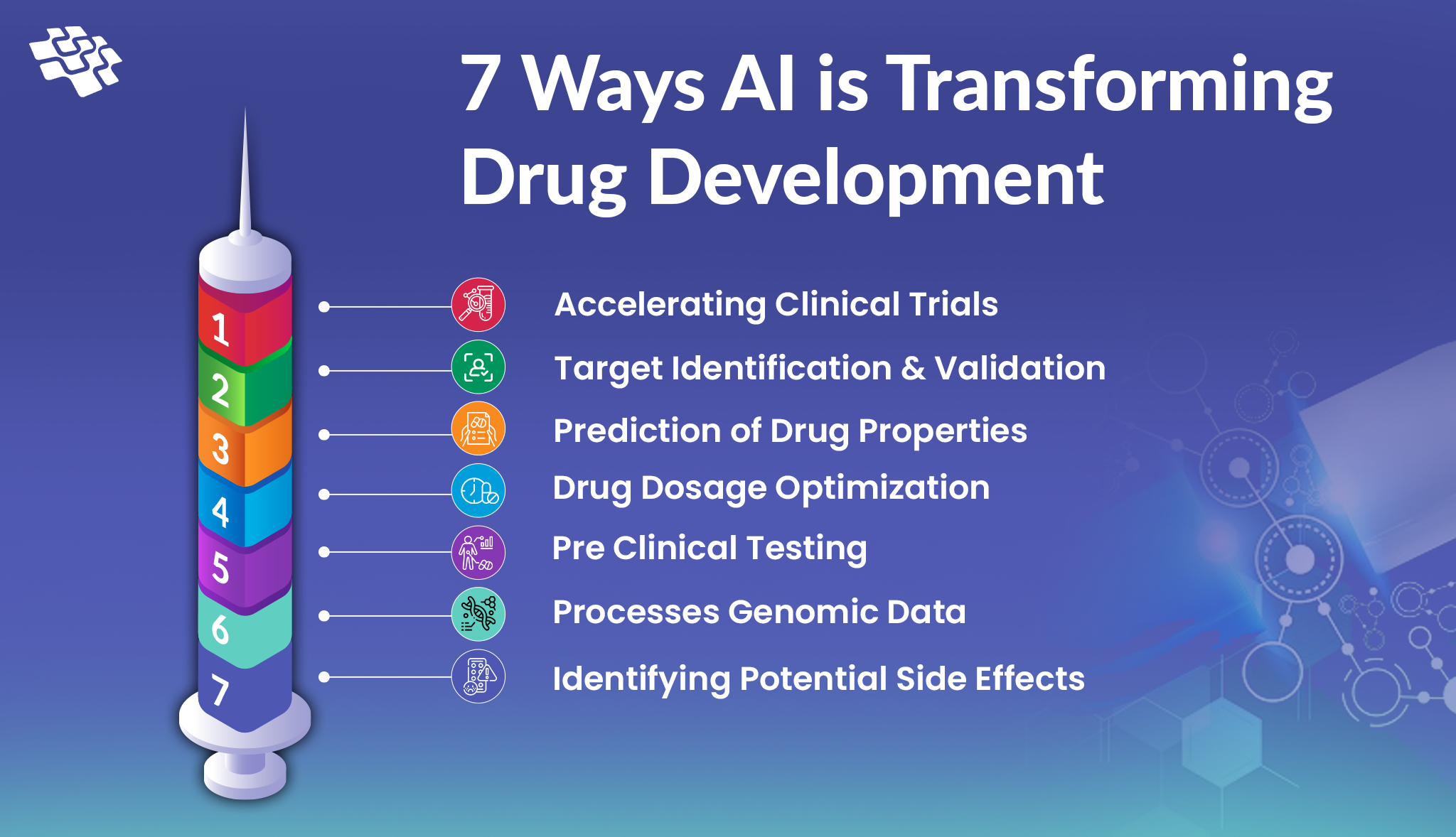
How AI in Drug Development is Transforming the Industry?
When trying to make an appointment for a medical visit, have you ever found that you have to wait weeks for the next open time slot? Everyone has experienced that. Mobile apps, however, have completely changed how we make appointments...
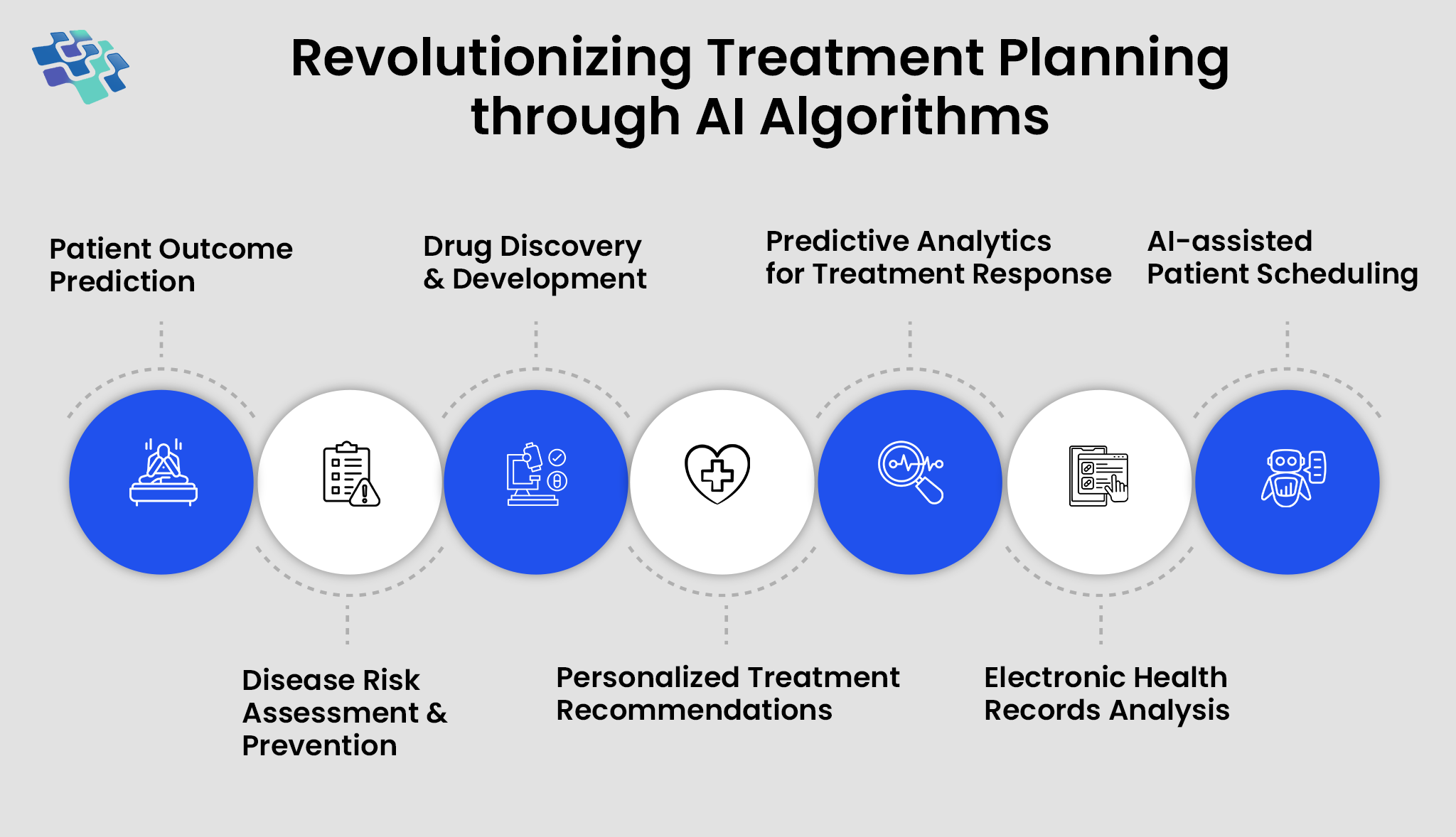
The Role of AI in Personalized Healthcare
What the American Medical Association refers to as AI’s “augmented intelligence” role is improving healthcare through better diagnostics, tailored medication, patient involvement, and dismantling data silos to transform patient care...
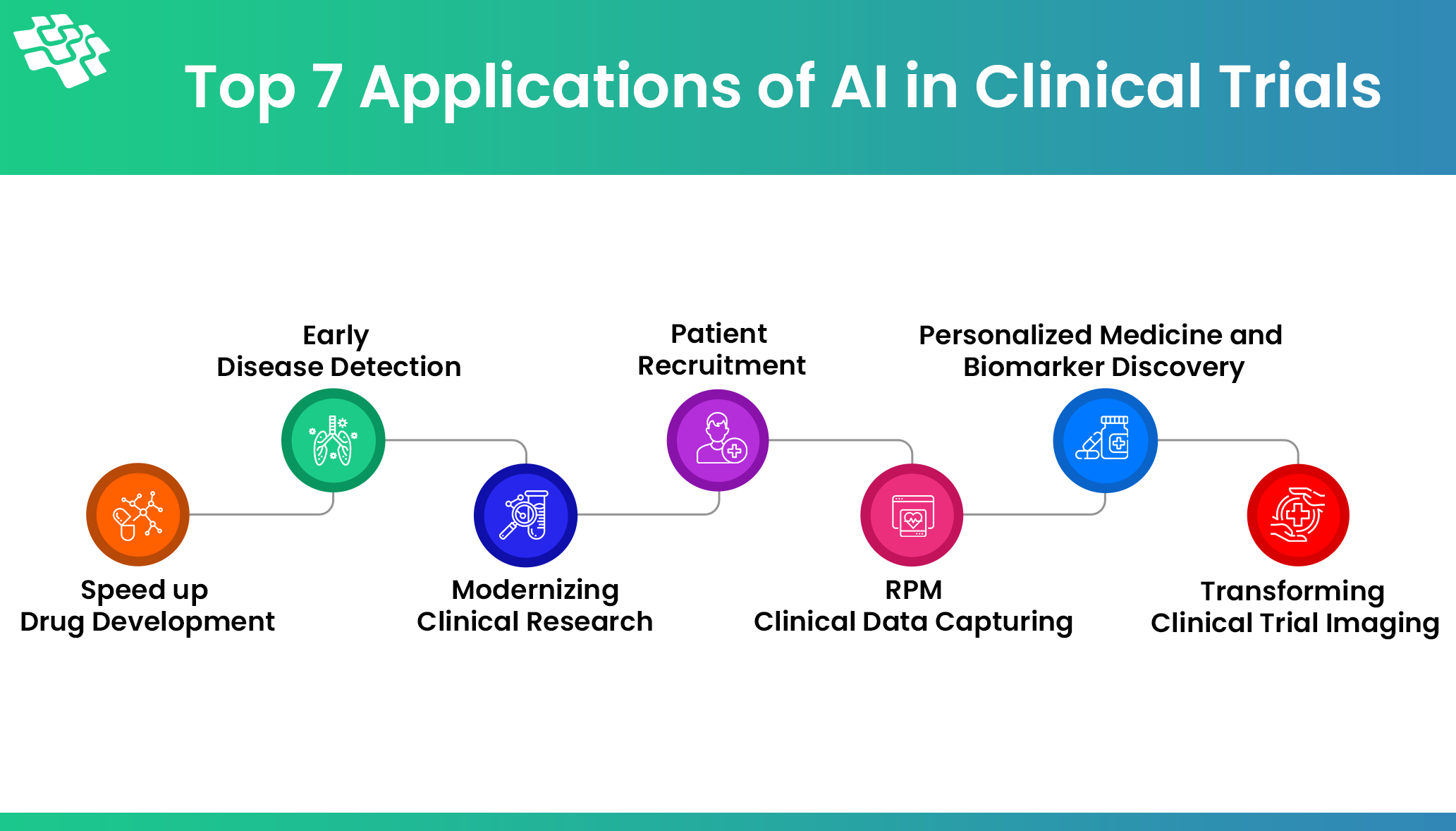
How AI in Clinical Trials is Improving Patient Outcomes?
The past year has seen a lot of coverage of AI because of its potential applications in business, logistics, technology, healthcare, and other fields. What about clinical research, though? Sponsors and clinical researchers should anticipate using AI in clinical trials.
Stay In the Know
Get Latest updates and industry insights every month.